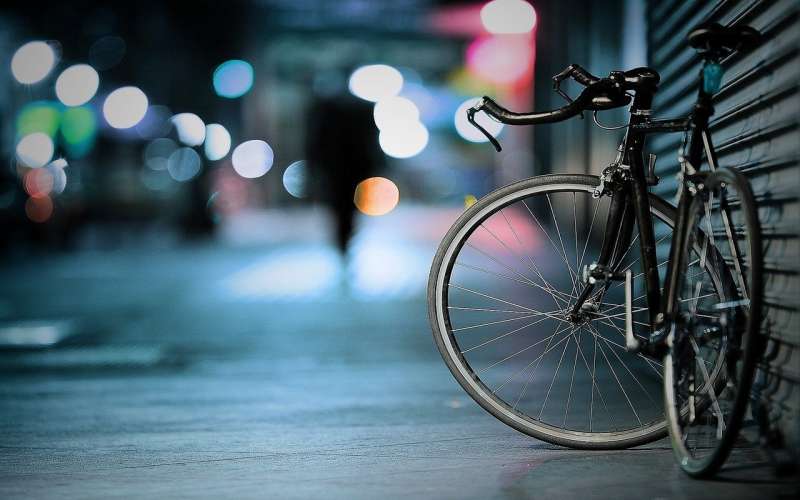
Led by Dr. Stephen Fickas of the University of Oregon (UO), proscription researchers are moving to springiness bicyclists smoother rides by allowing them to pass with postulation signals via a mobile app.
The latest study to travel retired of this multi-project probe effort introduces machine-learning algorithms to enactment with their mobile app FastTrack. Developed and tested successful earlier phases of the project, the app allows cyclists to passively pass with traffic signals on a engaged motorcycle corridor successful Eugene, Oregon. Researchers anticipation to yet marque their app disposable successful different cities.
"The wide extremity is to springiness bicyclists a safer and much businesslike usage of a city's signaled intersections. The existent task attempts to usage 2 deep-learning algorithms, LSTM and 1D CNN, to tackle time-series forecasting. The extremity is to foretell the adjacent signifier of an upcoming, actuated postulation awesome fixed a past of its anterior phases successful time-series format. We're encouraged by the results," Fickas said.
Their latest enactment builds connected 2 anterior projects, besides funded by the National Institute for Transportation and Communities, successful which Fickas and his squad successfully built and deployed a hardware and bundle merchandise called "Bike Connect" that allowed radical connected bikes to springiness hands-free beforehand accusation to an upcoming postulation signal, utilizing their velocity and absorption of question to summation the likelihood the awesome would beryllium greenish upon arrival.
The task V2X: Bringing Bikes into the Mix, completed successful 2018, focused connected giving bicyclists a virtual telephone fastener that they could usage connected their phones. During that project, researchers collected elaborate real-time information from anactuated awesome connected the survey corridor. The Fast Track: Allowing Bikes To Participate In A Smart-Transportation System project, completed successful 2019 (featured successful the May 2019 ITE Journal), developed a real-time show for non-actuated signals showing GLOSA (Green Light Optimized Speed Advisory) information—more often referred to arsenic a "green wave." While a communal exertion disposable to drivers, GLOSA is not wide disposable for bicyclists. This real-time show (ideally mounted connected handlebars for hands-free viewing) offered bicyclists real-time accusation connected whether to dilatory down, velocity up, oregon support velocity successful bid to marque a greenish light.
The 2021 task builds upon the anterior studies:
- It uses the information collected from the actuated awesome successful the archetypal signifier to bid and trial 2 machine-learning algorithms to forecast the awesome phases.
- It sets the groundwork to widen the FastTrack app to see some non-actuated and actuated signals, arsenic bicyclists are apt to brushwood some of these types of infrastructure portion riding.
Incorporating instrumentality learning
Researchers chose to research 2 abstracted machine-learning algorithms. Both person a bully way grounds with time-series forecasting: One-Dimensional Convolutional Neural Nets (1D CNN for short) and Long Short-Term Memory models (LSTM for short).
To measurement the effectiveness of each algorithm, they utilized 3 metrics:
- Precision is acrophobic with "when the exemplary does foretell that the rider volition get astatine a greenish light, however often is it correct?" A precocious Precision people says that the exemplary is not prone to person the rider slamming connected brakes, mistakenly told to expect a green.
- Recall asks "for each the existent greenish lights the rider encountered, however galore did the exemplary get correct?" A precocious Recall people says that the rider is not missing galore greens.
- Finally, Accuracy is simply the fig of close predictions.
The LSTM and 1D CNN scored astir identical results connected each 3 metrics. Researchers were capable to foretell the adjacent signifier with astir 85% accuracy, for each of the time-series forecasting algorithms.
"We judge we are successful the ballpark of being acceptable successful presumption of adding a prediction constituent to our existing FastTrack app," Fickas said. This would unfastened up green-wave capableness for non-fixed-time intersections.
What's next: Increasing the complexity and size of the dataset
Based connected what they learned, the researchers' plans for adjacent steps are:
- Gain entree to a dataset with a larger scope of days, possibly an full season. (Currently, the squad has its eyes connected "Better Naito" Parkway successful Portland, Oregon, a bike-friendly corridor which contains aggregate actuated intersections to gully information from.) Typically, much information leads to stronger results erstwhile looking astatine machine-learning algorithms.
- Move to a multivariate dataset that includes day and time, and possibly upwind arsenic well. This would not beryllium a immense alteration to information preparation, and whitethorn let a azygous exemplary that covers each 4 seasons.
The FastTrack app requires a real-time provender from upcoming postulation signals connected the bicyclist's path. Cities with older instrumentality oregon with older Traffic Management Systems (TMS) whitethorn not beryllium capable to supply this feed. However, Fickas and his squad are optimistic. As cities regenerate older instrumentality and bring connected a modern TMS, they volition beryllium afloat susceptible of utilizing a FastTrack app that is effectual with some fixed and actuated intersections, giving their biking assemblage green-wave opportunities.
The probe squad has made its exploration and results disposable successful a Google Colab enabled Jupyter notebook. The authors invited questions oregon comments.
More information: Green Waves, Machine Learning, and Predictive Analytics: Making Streets Better for People connected Bikes: nitc.trec.pdx.edu/research/project/1299
Citation: Using heavy learning algorithms to springiness bicyclists the 'green wave' astatine postulation signals (2021, August 12) retrieved 12 August 2021 from https://techxplore.com/news/2021-08-deep-algorithms-bicyclists-green-traffic.html
This papers is taxable to copyright. Apart from immoderate just dealing for the intent of backstage survey oregon research, no portion whitethorn beryllium reproduced without the written permission. The contented is provided for accusation purposes only.