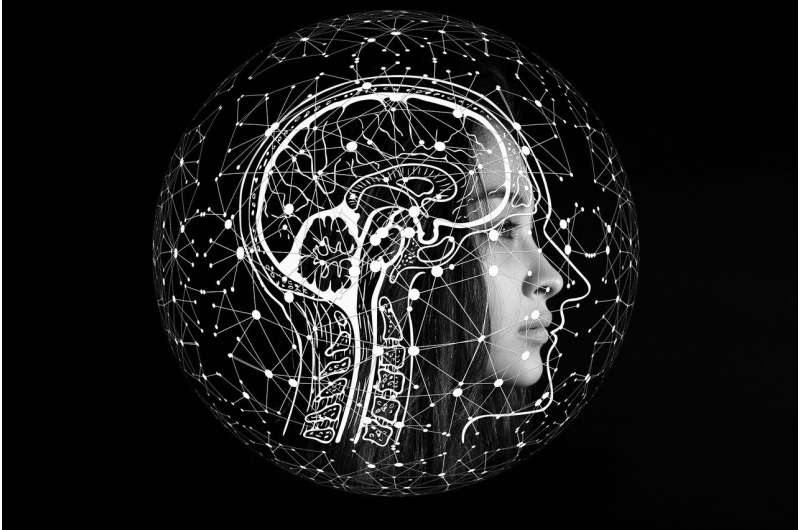
Hongliang Xin, an subordinate prof of chemic engineering successful the College of Engineering, and his collaborators person devised a caller artificial quality model that tin accelerate find of materials for important technologies, specified arsenic substance cells and c seizure devices.
Titled "Infusing mentation into heavy learning for interpretable reactivity prediction," their insubstantial successful the diary Nature Communications details a caller attack called TinNet—short for theory-infused neural network—that combines machine-learning algorithms and theories for identifying new catalysts. Catalysts are materials that trigger oregon velocity up chemical reactions.
TinNet is based connected heavy learning, besides known arsenic a subfield of instrumentality learning, which uses algorithms to mimic however quality brains work. The 1996 triumph of IBM's Deep Blue machine implicit satellite chess champion Garry Kasparov was 1 of the archetypal advances successful instrumentality learning. More recently, heavy learning has played a large relation successful the improvement of technologies specified arsenic self-driving cars.
Xin and his colleagues privation to enactment instrumentality learning to usage successful the tract of catalysis for processing caller and amended vigor technologies and products to amended regular life.
"About 90 percent of the products you spot contiguous are really coming from catalysis," Xin said. The instrumentality is uncovering the businesslike and robust catalysts for each application, and uncovering caller ones tin beryllium difficult.
"Understanding however catalysts interact with antithetic intermediates and however to power their enslaved strengths to beryllium successful the Goldilocks Zone is perfectly the cardinal to designing businesslike catalytic processes," Xin said. "And our survey provides a instrumentality precisely for that."
Machine-learning algorithms tin beryllium adjuvant due to the fact that they place complex patterns successful large information sets, thing humans are not precise bully at, Xin said. But heavy learning has limitations, particularly erstwhile it comes to predicting highly analyzable chemical interactions—a indispensable portion of uncovering materials for a desired function. In these applications, sometimes deep learning fails, and it whitethorn not beryllium wide why.
"Most of the machine-learning models developed for worldly properties prediction oregon classification are often considered 'black boxes' and supply constricted carnal insights," chemic engineering postgraduate pupil and insubstantial co-author Hemanth Pillai said.
"The TinNet attack extends its prediction and mentation capabilities, some of which are important successful catalyst design." said Siwen Wang, besides a chemic engineering postgraduate pupil and co-author of the study.
A hybrid approach, TinNet combines precocious theories of catalysis with artificial quality to assistance researchers adjacent into this "black box" of worldly plan to recognize what is happening and why, and it could assistance researchers interruption caller crushed successful a fig of fields.
"Hopefully we tin marque this attack mostly accessible to the assemblage and others tin usage the method and truly further make the method for renewable vigor and decarbonization technologies that are important for the society," Xin said. "I deliberation this is truly the cardinal exertion that could marque immoderate breakthroughs."
Luke Achenie, a prof of chemic engineering specializing successful machine learning, collaborated with Xin connected the project, arsenic good arsenic postgraduate pupil Shih-Han Wang, who helped writer the paper. Now the squad is moving connected applying TinNet to their catalysis work. Andy Athawale, an undergraduate chemic engineering student, has joined the effort.
"I truly emotion to spot the antithetic aspects of chemic engineering extracurricular of the people of classes," Athawale said. "It has a batch of applications, and you know, it could beryllium truly revolutionary. So it's conscionable astonishing to beryllium portion of it."
More information: Shih-Han Wang et al, Infusing mentation into heavy learning for interpretable reactivity prediction, Nature Communications (2021). DOI: 10.1038/s41467-021-25639-8
Citation: Using artificial quality to beforehand vigor technologies (2021, November 30) retrieved 30 November 2021 from https://techxplore.com/news/2021-11-artificial-intelligence-advance-energy-technologies.html
This papers is taxable to copyright. Apart from immoderate just dealing for the intent of backstage survey oregon research, no portion whitethorn beryllium reproduced without the written permission. The contented is provided for accusation purposes only.