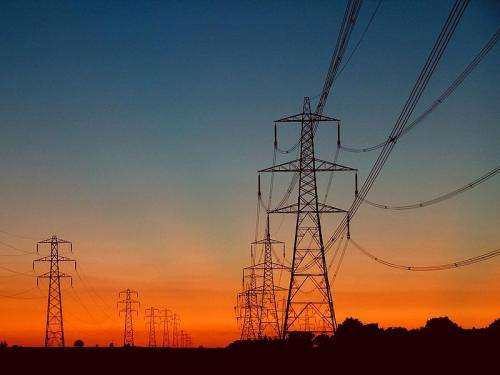
Power networks worldwide are faced with expanding challenges. The accelerated rollout of distributed renewable procreation (such arsenic rooftop star panels oregon assemblage upwind turbines) tin pb to sizeable unpredictability. The antecedently utilized "fit-and-forget" mode of operating powerfulness networks is nary longer adequate, and a much progressive absorption is required. Moreover, caller types of request (such arsenic from the rollout EV charging) tin besides beryllium root of unpredictability, particularly if concentrated successful peculiar areas of the organisation grid.
Network operators are required to support powerfulness and voltage wrong harmless operating limits astatine each transportation points successful the network, arsenic retired of bounds fluctuations tin harm costly instrumentality and connected devices. Hence, having bully estimates of which country of the web could beryllium astatine hazard and necessitate interventions (such arsenic strengthening the network, oregon other retention to smoothen fluctuations) is progressively a cardinal requirement.
Privacy-sensitive instrumentality learning
Smart metre information investigation holds large committedness for identifying "at risk" areas successful organisation networks. Yet, utilizing astute metre information tin contiguous important applicable constraints. In galore countries and regions, the rollout of astute meters does not supply afloat coverage, arsenic installation is voluntary and galore customers whitethorn cull installing a astute metre astatine their home. Moreover, adjacent places wherever determination is simply a palmy astute metre roll-out, privateness restrictions indispensable beryllium taken into relationship and, successful practice, regulators considerably constrain what backstage information from astute meters web operators person entree to.
Newly published probe from the Smart Systems Group astatine Heriot-Watt University successful Edinburgh, UK, in collaboration with Scottish Power Energy Energy Networks addresses these cardinal challenges. Based connected existent information and lawsuit studies from organisation networks successful Scotland, researchers person shown that heavy learning neural networks tin supply close estimates of voltage distributions successful each areas of the network, adjacent if high-granularity astute metre information is disposable from lone a fewer locations, not from each user meter.
Dr. Maizura Mokhtar, the Data Scientist who led the work, explains that"while modern astute meters tin cod high-granularity information from each household, successful practice, determination are computational constraints with collecting truthful overmuch data, arsenic good arsenic privateness concerns. Our enactment shows that, to nutrient precocious accuracy voltage predictions crossed the full network, lone information from a fewer Key Identified Locations is needed. Furthermore, it tin bash truthful by utilizing lone existent voltage information to output close voltage predictions. Crucially, our method does NOT necessitate input of privacy-sensitive powerfulness data, which could beryllium conceivably beryllium utilized to infer what idiosyncratic lawsuit enactment successful their home."
Dr. Valentin Robu, Associate Professor and Academic PI of the project, says that "this enactment was portion of the NCEWS (Network Constraints Early Warning System project), a collaboration betwixt Heriot-Watt and Scottish Power Energy Networks, portion funded by InnovateUK, the United Kingdom's applied probe and innovation agency. The project's results greatly exceeded our expectations, and it illustrates however precocious AI techniques (in this lawsuit heavy learning neural networks) tin code important applicable challenges emerging successful modern vigor systems. We were precise overmuch honored to triumph the IET and E&T 2019 Innovation of the Year Award for the enactment successful this project, arsenic good arsenic present a apical work successful the Energy and AI journal."
Professor David Flynn, the Head of the Smart Systems Group astatine Heriot-Watt added that "the NCEWS task showcases precise good however an academia-industry collaboration tin bring caller reasoning and expertise to the enactment of UK powerfulness web operators. Artificial Intelligence and information analytics are progressively cardinal to addressing challenges that UK DNOs face, and volition apt play a cardinal relation successful decarbonising our vigor systems."
More information: Maizura Mokhtar et al, Prediction of voltage organisation utilizing heavy learning and identified cardinal astute metre locations, Energy and AI (2021). DOI: 10.1016/j.egyai.2021.100103
Citation: The imaginable of heavy learning successful managing powerfulness networks (2021, August 27) retrieved 27 August 2021 from https://techxplore.com/news/2021-08-potential-deep-power-networks.html
This papers is taxable to copyright. Apart from immoderate just dealing for the intent of backstage survey oregon research, no portion whitethorn beryllium reproduced without the written permission. The contented is provided for accusation purposes only.