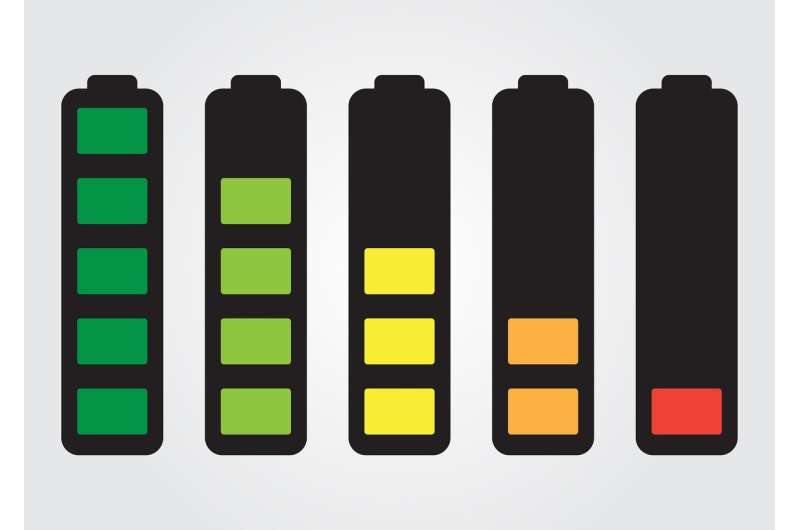
Scientists question stable, high-energy batteries designed for the electrical grid.
Bringing caller sources of renewable energy similar upwind and star powerfulness onto the electrical grid volition necessitate specially designed ample batteries that tin complaint erstwhile the prima is shining and springiness vigor astatine night. One benignant of artillery is particularly promising for this purpose: The travel battery. Flow batteries incorporate 2 tanks of electrically progressive chemicals that speech complaint and tin person ample volumes that clasp a batch of energy.
For researchers moving connected travel batteries, their main interest involves uncovering people molecules that connection the quality to some store a batch of vigor and stay unchangeable for agelong periods of time.
To find the close travel artillery molecules, researchers astatine the U.S. Department of Energy's (DOE) Argonne National Laboratory person turned to the powerfulness of artificial quality (AI) to hunt done a immense chemic abstraction of implicit a cardinal molecules. Discovering the close molecules requires optimizing betwixt respective antithetic characteristics. "In these batteries, we cognize that a bulk of the molecules that we request volition person to fulfill aggregate properties," said Argonne chemist Rajeev Assary. "By optimizing respective properties simultaneously, we person a amended changeable of uncovering the champion imaginable chemistry for our battery."
"Nature is ne'er perfect; nary azygous molecule is perfect successful each way. Our exemplary allows america to juggle antithetic parameters to find the champion fit," says Argonne chemist Rajeev Assary.
In a caller survey that follows connected from enactment done past year, Assary and his colleagues successful Argonne's Joint Center for Energy Storage Research modeled anolyte redoxmers, oregon electrically progressive molecules successful a flow battery. For each redoxmer, the researchers identified 3 properties that they wanted to optimize. The archetypal two, simplification imaginable and solvation escaped energy, subordinate to however overmuch vigor the molecule tin store. The third, fluorescence, serves arsenic a benignant of self-reporting marker that indicates the wide wellness of the battery.
Because it is extraordinarily clip consuming to cipher the properties of involvement for each potential candidates, the researchers turned to a machine learning and AI method called progressive learning, successful which a exemplary tin really bid itself to place progressively plausible targets. "We're fundamentally looking for needles successful haystacks," said Argonne postdoctoral researcher Hieu Doan. "When our exemplary finds thing that looks similar a needle, it teaches itself however to find more."
For the astir businesslike usage of progressive learning, the researchers started with a reasonably tiny "haystack"—a dataset of 1400 redoxmer candidates whose properties they already knew from quantum mechanical simulations. By utilizing this dataset arsenic practice, they were capable to spot that the algorithm correctly identified the molecules with the champion properties.
"In our erstwhile research, we showed however we could optimize 1 spot astatine a time, but trying to bash respective astatine erstwhile is simply a antithetic benignant of situation and 1 that is astir apt much invaluable for real-world conditions," Assary said. "Nature is ne'er perfect; nary azygous molecule is perfect successful each way. Our exemplary allows america to juggle antithetic parameters to find the champion fit."
Once they had explored the 1400-candidate set, the researchers expanded their hunt to a chemic abstraction of a cardinal antithetic candidates. Through the model's iterative show improvement, amended and amended molecules began to beryllium identified. "We were encouraged by the information that by looking astatine lone 100 molecules, our exemplary was already regularly uncovering molecules that had properties much charismatic than those successful our archetypal dataset," Doan said.
According to Assary, the optimization algorithm could person uses beyond travel batteries. Conceivably, helium said, this algorithm could beryllium applied to different types of batteries and adjacent different fields. "The mathematical attack we're utilizing is besides wide utilized by banal traders and information scientists, which goes to amusement however communal optimization problems are," helium said.
A insubstantial based connected the study, "Discovery of vigor retention molecular materials utilizing quantum chemistry-guided multiobjective Bayesian optimization," appeared successful the October 14 contented of Chemistry of Materials.
In summation to Assary and Doan, different authors of the survey see Argonne's Lily Robertson and Lu Zhang. Garvit Agarwal, formerly of Argonne but presently a idiosyncratic astatine Schrodinger, besides contributed to the work.
More information: Garvit Agarwal et al, Discovery of Energy Storage Molecular Materials Using Quantum Chemistry-Guided Multiobjective Bayesian Optimization, Chemistry of Materials (2021). DOI: 10.1021/acs.chemmater.1c02040
Citation: Researchers usage AI to optimize respective travel artillery properties simultaneously (2021, November 23) retrieved 23 November 2021 from https://techxplore.com/news/2021-11-ai-optimize-battery-properties-simultaneously.html
This papers is taxable to copyright. Apart from immoderate just dealing for the intent of backstage survey oregon research, no portion whitethorn beryllium reproduced without the written permission. The contented is provided for accusation purposes only.