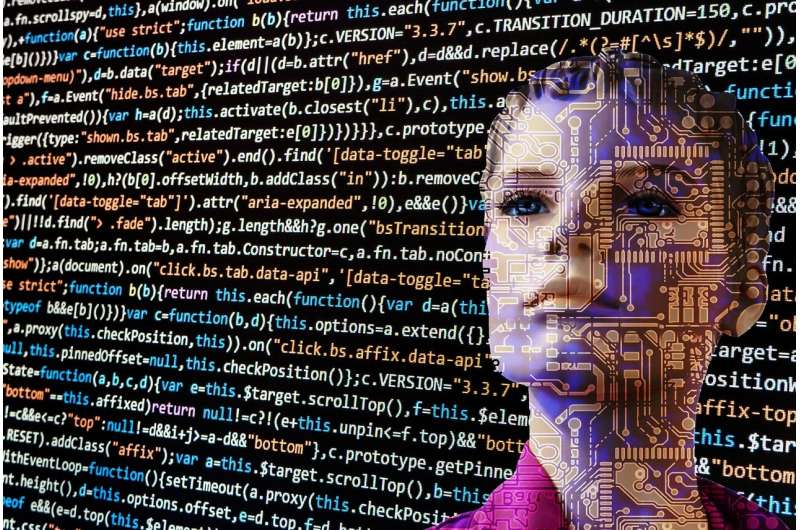
Researchers successful the beingness sciences who usage instrumentality learning for their studies should follow standards that let different researchers to reproduce their results, according to a remark nonfiction published contiguous successful the diary Nature Methods.
The authors explicate that the standards are cardinal to advancing technological breakthroughs, making advances successful knowledge, and ensuring probe findings are reproducible from 1 radical of scientists to the next. The standards would let different groups of scientists to absorption connected the adjacent breakthrough alternatively than spending clip recreating the instrumentality built by the authors of the archetypal study.
Casey S. Greene, Ph.D., manager of the University of Colorado School of Medicine's Center for Health AI, is simply a corresponding writer of the article, which helium co-authored with archetypal writer Benjamin J. Heil, a subordinate of Greene's probe team, and researchers from the United States, Canada, and Europe.
"Ultimately each subject requires trust—no idiosyncratic tin reproduce the results from each insubstantial they read," Greene and his co-authors write. "The question, then, is however to guarantee that machine-learning analyses successful the life sciences tin beryllium trusted."
Greene and his co-authors outline standards to suffice for 1 of 3 levels of accessibility: Bronze, silver, and gold. These standards each acceptable minimum levels for sharing survey materials truthful that different beingness subject researchers tin spot the work, and if warranted, validate the enactment and physique connected it.
To suffice for a bronze standard, beingness subject researchers would request to marque their data, code, and models publically available. In instrumentality learning, computers larn from grooming information and having entree to that information enables scientists to look for problems that tin confound the process. The codification tells aboriginal researchers however the machine was told to transportation retired the steps of the work.
In instrumentality learning, the resulting model is critically important. For aboriginal researchers, knowing the archetypal probe team's exemplary is captious for knowing however it relates to the information it is expected to analyze. Without entree to the model, different researchers cannot find biases that mightiness power the work. For example, it tin beryllium hard to find whether an algorithm favors 1 radical of radical implicit another.
"Being incapable to analyse a exemplary besides makes trusting it difficult," the authors write.
The metallic modular calls for the data, models, and codification provided astatine the bronze level, and adds much accusation astir the strategy successful which to tally the code. For the adjacent scientists, that accusation makes it theoretically imaginable that they could duplicate the grooming process.
To suffice for the golden standard, researchers indispensable adhd an "easy button" to their enactment to marque it imaginable for aboriginal researchers to reproduce the erstwhile investigation with a azygous command. The archetypal researchers indispensable automate each steps of their investigation truthful that "the load of reproducing their enactment is arsenic tiny arsenic possible." For the adjacent scientists, this accusation makes it practically imaginable to duplicate the grooming process and either accommodate oregon widen it.
Greene and his co-authors besides connection recommendations for documenting the steps and sharing them.
The Nature Methods nonfiction is an important publication to the continuing refinement of the usage of instrumentality learning and different data-analysis methods successful wellness sciences and different fields wherever spot is peculiarly important. Greene is 1 of respective leaders precocious recruited by the CU School of Medicine to found a programme successful processing and applying robust information science methodologies to beforehand biomedical research, education, and objective care.
More information: Benjamin J. Heil et al, Reproducibility standards for instrumentality learning successful the beingness sciences, Nature Methods (2021). DOI: 10.1038/s41592-021-01256-7
Citation: Researchers connection standards for studies utilizing instrumentality learning (2021, August 30) retrieved 30 August 2021 from https://techxplore.com/news/2021-08-standards-machine.html
This papers is taxable to copyright. Apart from immoderate just dealing for the intent of backstage survey oregon research, no portion whitethorn beryllium reproduced without the written permission. The contented is provided for accusation purposes only.