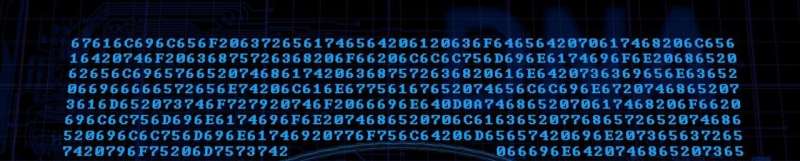
Machine-learning algorithms are utilized to find patterns successful information that humans wouldn't different notice, and are being deployed to assistance pass decisions large and small—from COVID-19 vaccination improvement to Netflix recommendations.
New award-winning probe from the Cornell Ann S. Bowers College of Computing and Information Science explores however to assistance nonexperts effectively, efficiently and ethically usage machine-learning algorithms to amended alteration industries beyond the computing tract to harness the powerfulness of AI.
"We don't cognize overmuch astir however nonexperts successful machine learning travel to larn algorithmic tools," said Swati Mishra, a Ph.D. pupil successful the tract of information science. "The crushed is that there's a hype that's developed that suggests instrumentality learning is for the ordained."
Mishra is pb writer of "Designing Interactive Transfer Learning Tools for ML Non-Experts," which received a Best Paper Award astatine the yearly ACM CHI Virtual Conference connected Human Factors successful Computing Systems, held successful May.
As instrumentality learning has entered fields and industries traditionally extracurricular of computing, the request for probe and effective, accessible tools to alteration caller users successful leveraging artificial quality is unprecedented, Mishra said.
Existing probe into these interactive machine-learning systems has mostly focused connected knowing the users and the challenges they look erstwhile navigating the tools. Mishra's latest research—including the improvement of her ain interactive machine-learning platform—breaks caller crushed by investigating the inverse: How to amended plan the strategy truthful that users with constricted algorithmic expertise but immense domain expertise tin larn to integrate preexisting models into their ain work.
"When you bash a task, you cognize what parts request manual fixing and what needs automation," said Mishra, a 2021-2022 Bloomberg Data Science Ph.D. fellow. "If we plan machine-learning tools correctly and springiness capable bureau to radical to usage them, we tin guarantee their cognition gets integrated into the machine-learning model."
Mishra takes an unconventional attack with this probe by turning to a analyzable process called "transfer learning" arsenic a jumping-off constituent to initiate nonexperts into instrumentality learning. Transfer learning is simply a high-level and almighty machine-learning method typically reserved for experts, wherein users repurpose and tweak existing, pretrained machine-learning models for caller tasks.
The method alleviates the request to physique a exemplary from scratch, which requires tons of grooming data, allowing the idiosyncratic to repurpose a exemplary trained to place images of dogs, say, into a exemplary that tin place cats or, with the close expertise, adjacent tegument cancers.
"By intentionally focusing connected appropriating existing models into caller tasks, Swati's enactment helps novices not lone usage instrumentality learning to lick complex tasks, but besides instrumentality vantage of machine-learning experts' continuing developments," said Jeff Rzeszotarski, adjunct prof successful the Department of Information Science and the paper's elder author. "While our eventual extremity is to assistance novices go precocious machine-learning users, providing immoderate 'training wheels' done transportation learning tin assistance novices instantly employment instrumentality learning for their ain tasks."
Mishra's probe exposes transportation learning's interior computational workings done an interactive level truthful nonexperts tin amended recognize however machines crunch datasets and marque decisions. Through a corresponding laboratory survey with radical with nary inheritance successful machine-learning development, Mishra was capable to pinpoint precisely wherever beginners mislaid their way, what their rationales were for making definite tweaks to the model and what approaches were astir palmy oregon unsuccessful.
In the end, the duo recovered participating nonexperts were capable to successfully usage transportation learning and change existing models for their ain purposes. However, researchers discovered that inaccurate perceptions of instrumentality quality often slowed learning among nonexperts. Machines don't larn similar humans do, Mishra said.
"We're utilized to a human-like learning style, and intuitively we thin to employment strategies that are acquainted to us," she said. "If the tools bash not explicitly convey this difference, the machines whitethorn ne'er truly learn. We arsenic researchers and designers person to mitigate idiosyncratic perceptions of what instrumentality learning is. Any interactive tool indispensable assistance america negociate our expectations."
More information: Swati Mishra et al, Designing Interactive Transfer Learning Tools for ML Non-Experts, Proceedings of the 2021 CHI Conference connected Human Factors successful Computing Systems (2021). DOI: 10.1145/3411764.3445096
Citation: Platform teaches nonexperts to usage instrumentality learning (2021, July 30) retrieved 30 July 2021 from https://techxplore.com/news/2021-07-platform-nonexperts-machine.html
This papers is taxable to copyright. Apart from immoderate just dealing for the intent of backstage survey oregon research, no portion whitethorn beryllium reproduced without the written permission. The contented is provided for accusation purposes only.