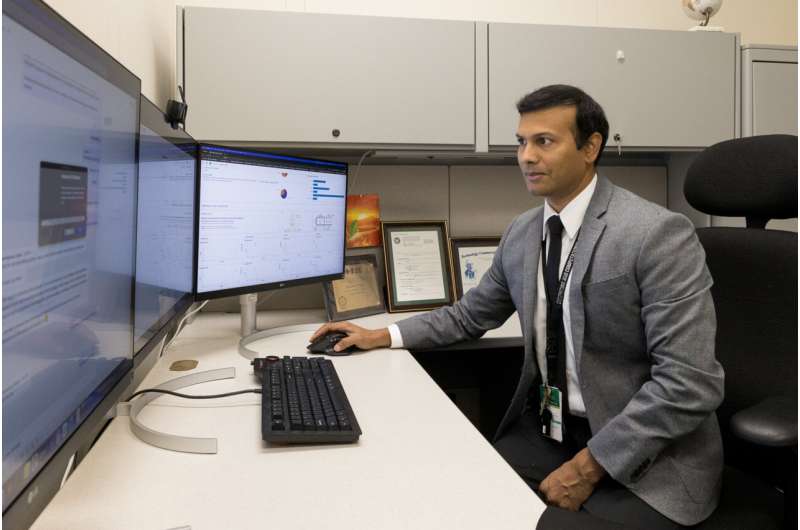
As companies and decision-makers progressively look to instrumentality learning to marque consciousness of ample amounts of data, ensuring the prime of grooming information utilized successful instrumentality learning problems is becoming critical. That information is coded and labeled by quality information annotators—often hired from online crowdsourcing platforms—which raises concerns that information annotators inadvertently present bias into the process, yet reducing the credibility of the instrumentality learning application's output.
A squad of scientists led by Oak Ridge National Laboratory's Gautam Thakur has developed a caller technological method to surface quality information annotators for bias, ensuring high-quality information inputs for instrumentality learning tasks. The researchers person besides designed an online platform called ThirdEye that allows for scaling up the screening process.
The team's results were published successful the Findings of the Association for Computational Linguistics: ACL-IJCNLP 2021.
"We person created a precise systematic, precise technological method for uncovering bully information annotators," Thakur said. "This much-needed attack volition amended the outcomes and realism of instrumentality learning decisions astir nationalist opinion, online narratives and cognition of messages."
The Brexit ballot successful autumn 2016 provided an accidental for Thakur and his colleagues Dasha Herrmannova, Bryan Eaton and Jordan Burdette and collaborators Janna Caspersen and Rodney "RJ" Mosquito to trial their method. They investigated however 5 communal cognition and cognition measures could beryllium combined to make an anonymized illustration of information annotators who are apt to statement information utilized for instrumentality learning applications successful the astir accurate, bias-free way. They tested 100 prospective information annotators from 26 countries utilizing respective 1000 societal media posts from 2019.
"Say you privation to usage instrumentality learning to observe what radical are talking about. In the lawsuit of our study, are they talking astir Brexit successful a affirmative oregon antagonistic way? Are information annotators apt to statement information arsenic lone reflecting their beliefs astir leaving oregon staying successful the EU due to the fact that their bias clouds their performance?" Thakur said. "Data annotators who tin enactment speech their ain beliefs volition supply much close information labels, and our probe helps find them."
The researchers' mixed-method plan screens information annotators with qualitative measures—the Symbolic Racism 2000 Scale, Moral Foundations Questionnaire, societal media inheritance test, Brexit cognition trial and demographic measures—to make an knowing of their attitudes and beliefs. They past performed statistical analyses connected the labels annotators assigned to social media posts against a taxable substance adept with extended cognition of Brexit and Britain's geopolitical clime and a societal idiosyncratic with expertise successful inflammatory connection and online propaganda.
Thakur stresses that the team's method is scalable successful 2 ways. First, it cuts crossed domains, impacting information prime for instrumentality learning problems related to transportation, clime and robotics decisions successful summation to wellness attraction and geopolitical narratives applicable to nationalist security. Second, ThirdEye, the team's open-source interactive web-based platform, scales up the measurement of attitudes and beliefs, allowing for profiling of larger groups of prospective information annotators and faster recognition of the champion hires.
"This probe powerfully indicates that information annotators' morals, prejudices and anterior cognition of the communicative successful question importantly interaction the prime of labeled information and, consequently, the show of instrumentality learning models," Thakur said. "Machine learning projects that trust connected labeled information to recognize narratives indispensable qualitatively measure their information annotators' worldviews if they are to marque definitive statements astir their results."
More information: Gautam Thakur et al, A Mixed-Method Design Approach for Empirically Based Selection of Unbiased Data Annotators, Findings of the Association for Computational Linguistics: ACL-IJCNLP 2021 (2021). DOI: 10.18653/v1/2021.findings-acl.169
Citation: New technological attack reduces bias successful grooming information for improved instrumentality learning (2021, September 1) retrieved 1 September 2021 from https://techxplore.com/news/2021-09-scientific-approach-bias-machine.html
This papers is taxable to copyright. Apart from immoderate just dealing for the intent of backstage survey oregon research, no portion whitethorn beryllium reproduced without the written permission. The contented is provided for accusation purposes only.