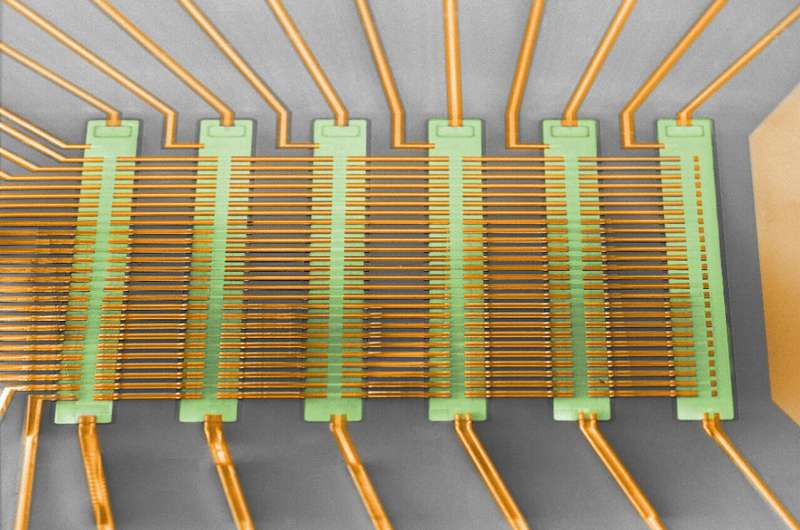
To bid and instrumentality artificial neural networks, engineers necessitate precocious devices susceptible of performing data-intensive computations. In caller years, probe teams worldwide person been trying to make specified devices, utilizing antithetic approaches and designs.
One imaginable mode to make these devices is to recognize specialized hardware onto which neural networks tin beryllium mapped directly. This could entail, for instance, the usage of arrays of memristive devices, which simultaneously execute parallel computations.
Researchers astatine Max Planck Institute of Microstructure Physics and the startup SEMRON GmbH successful Germany person precocious designed caller energy-efficient memcapacitive devices (i.e., capacitors with a memory) that could beryllium utilized to instrumentality machine-learning algorithms. These devices, presented successful a insubstantial published successful Nature Electronics, enactment by exploiting a rule known arsenic complaint shielding.
"We noticed that too accepted integer approaches for moving neural networks, determination were mostly memristive approaches and lone precise fewer memcapacitive proposals," Kai-Uwe Demasius, 1 of the researchers who carried retired the study, told TechXplore. "In addition, we noticed that each commercially disposable AI Chips are lone digital/mixed awesome based and determination are fewer chips with resistive representation devices. Therefore, we started to analyse an alternate attack based connected a capacitive representation device."
While reviewing erstwhile studies, Demasius and his colleagues observed that each existing memcapacitive devices were hard to standard up and exhibited a mediocre dynamic range. They frankincense acceptable retired to make devices that are much businesslike and easier to standard up. The caller memcapacitive instrumentality they created draws inspiration from synapses and neurotransmitters successful the brain.
"Memcapacitor devices are inherently galore times much vigor businesslike compared to memristive devices, due to the fact that they are electrical tract based alternatively of existent based and the signal-to-noise ratio is amended for the archetypal case," Demasius said. "Our memcapacitor instrumentality is based connected complaint screening, which enables overmuch amended scalability and higher dynamic scope successful examination to anterior trials to recognize memcapacitive devices."
The instrumentality created by Demasius and his colleagues controls the electric field coupling betwixt a apical gross electrode and a bottommost read-out electrode via different layer, called the shielding layer. This shielding furniture is successful crook adjusted by an analog memory, which tin store the antithetic value values of artificial neural networks, likewise to however neurotransmitters successful the encephalon store and convey information.
To measure their devices, the researchers arranged 156 of them successful a crossbar pattern, past utilized them to bid a neural web to separate betwixt 3 antithetic letters of the roman alphabet ("M," "P' and "I'). Remarkably, their devices attained vigor efficiencies of implicit 3,500 TOPS/W astatine 8 Bit precision, which is 35 to 300 times larger compared to different existing memresistive approaches. These findings item the imaginable of the team's caller memcapacitors for moving ample and analyzable heavy learning models with a precise debased powerfulness depletion (in the μW regime).
"We judge that the adjacent procreation human-machine interfaces volition heavy beryllium connected automatic code recognition (ASR)," Demasius said. "This not lone includes wake-up-word detection, but besides much analyzable algorithms, similar speech-to-text conversion. Currently ASR is mostly done successful the cloud, but processing connected the borderline has advantages with regards to information extortion amongst other."
If code designation techniques amended further, code could yet go the superior means done which users pass with computers and different physics devices. However, specified an betterment volition beryllium hard oregon intolerable to instrumentality without ample neural network-based models with billions of parameters. New devices that tin efficiently instrumentality these models, specified arsenic the 1 developed by Demasius and his colleagues, could frankincense play a important relation successful realizing the afloat imaginable of artificial quality (AI).
"We founded a start-up that facilitates this superior technology," Demasius said. "SEMRON´s imaginativeness is to alteration these ample artificial neural networks connected a precise tiny formfactor and powerfulness these algorithms with artillery powerfulness oregon adjacent vigor harvesting, for lawsuit connected receptor buds oregon immoderate different wearable."
SEMRON, the start-up founded by Demasius and his colleagues, has already applied for respective patents related to heavy learning models for code recognition. In the future, the squad plans to make much neural network-based models, portion besides trying to standard up the memcapacitor-based strategy they designed, by expanding some its ratio and device-density.
"We are perpetually filing patents for immoderate taxable related to this," Demasius said. "Our eventual extremity is to alteration each instrumentality to transportation heavy AI functionality connected instrumentality and we besides envision a batch of approaches erstwhile it comes to grooming oregon heavy learning exemplary architectures. Spiking neural nets and transformer based neural networks are lone immoderate examples. One spot is that we tin enactment each these approaches, but of people changeless probe is indispensable to support up with each caller concepts successful that domain."
More information: Kai-Uwe Demasius, Aron Kirschen, and Stuart Parkin, Energy-efficient memcapacitor devices for neuromorphic computing, Nature Electronics(2021). DOI: 10.1038/s41928-021-00649-y
© 2021 Science X Network
Citation: New memcapacitor devices for neuromorphic computing applications (2021, October 27) retrieved 27 October 2021 from https://techxplore.com/news/2021-10-memcapacitor-devices-neuromorphic-applications.html
This papers is taxable to copyright. Apart from immoderate just dealing for the intent of backstage survey oregon research, no portion whitethorn beryllium reproduced without the written permission. The contented is provided for accusation purposes only.