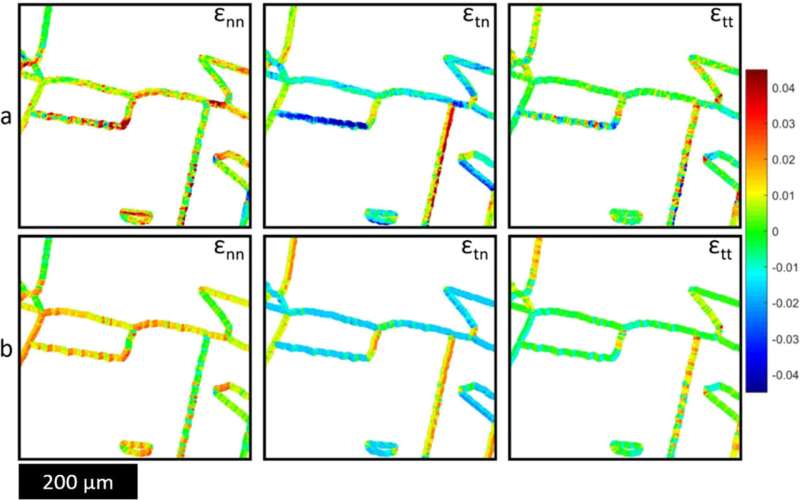
To the bare eye, a expanse of stainless alloy presents a smooth, polished, homogenous surface. The aforesaid worldly erstwhile viewed astatine 400 times magnification reveals its existent jumbled structure—different crystal shapes, joined astatine wildly antithetic angles. Researchers astatine the University of Illinois Urbana-Champaign utilized information from high-resolution images of stainless-steel samples to bid neural networks that marque predictions astir however the worldly volition behave astatine places wherever the crystals meet, erstwhile strained.
John Lambros explained, erstwhile studying the properties of a worldly specified arsenic stainless steel, it is intolerable to behaviour abstracted experiments astatine specified precocious magnifications that taxable it to each conceivable parameter—every temperature, each loading angle, each magnitude of pressure. So we often trust connected models.
"Rather than utilizing an highly elaborate and cumbersome physics model with a batch of fitting parameters, we utilized instrumentality learning to bid a neural web to marque these predictions," Lambros said. "Machine learning bypasses the request for a elaborate modeling of each of the physics down the process and makes a benignant of nonstop link, oregon fitting, betwixt the inputs and the outputs.
"This is the archetypal clip this method has been applied to larn what happens successful a metallic microstructure nether assorted loading conditions," Lambros said. "In this case, we wanted to spot however overmuch strain accumulated astatine atom boundaries successful a polycrystalline metallic during creep." He explained that creep is the inclination for a coagulated worldly to deform nether a continual load, overmuch similar immoderate bookshelves yet crook nether the value of the books.
Lambros and his postgraduate pupil Renato Vieira began the survey with an assumption.
"We believed the carnal differences betwixt the 2 grains adjacent to the bound would beryllium much important, oregon astatine slightest an arsenic important parameter. So, the astir singular uncovering for maine was that 1 azygous geometric parameter was capable to foretell the results 80 percent of the time," helium said. "It's the geometry —the space astatine which you're loading it that made the astir difference. I recovered that unexpected and interesting— not that it shouldn't power the results, but that it did truthful this dramatically. It was surprising, due to the fact that it means that each this sophisticated, multi-scale modeling that we bash to recognize each the physics whitethorn beryllium lone astir 20 percent important."
Lambros added that this is simply a preliminary study. "Being the archetypal of its kind, determination indispensable beryllium a batch much in-depth studying earlier we tin accidental this is universally true."
He noted that it works successful cases 70 to 80 percent of the time, but it doesn't foretell the effect of each the boundaries everywhere. "This means that determination are different factors beyond conscionable the space that are influencing what is happening. It's conscionable that this is the astir important one, oregon the archetypal bid one."
Lambros said helium wants to yet incorporated the technique, and what they've learned from it, into existing nonaccomplishment models.
"The instrumentality learning exemplary we person truthful acold lone works adjacent the grain boundaries," helium said. "We can't yet foretell what happens successful the atom interior. So, fig one, we request a antithetic acceptable of inputs that volition besides enactment successful the interior. We person them experimentally, but we request to make a antithetic exemplary and capable successful the gaps.
"Ultimately, what we privation is to beryllium capable to conscionable amusement an representation of the microstructure to the algorithm and the algorithm volition archer america erstwhile and wherever the worldly volition fail. But, alternatively than doing 1 large neural network acceptable from opening to end, we're going to bash steps successful betwixt that correspond the physics down it and wrong each measurement we volition marque usage of instrumentality learning to find the due inputs and outputs."
The study, "Machine Learning Neural-Network Predictions for Grain-Boundary Strain Accumulation successful a Polycrystalline Metal," was written by Renato Vieira and John Lambros. It is published successful Experimental Mechanics and besides appears arsenic the Journal's screen for the issue.
More information: R. B. Vieira et al, Machine Learning Neural-Network Predictions for Grain-Boundary Strain Accumulation successful a Polycrystalline Metal, Experimental Mechanics (2021). DOI: 10.1007/s11340-020-00687-1
Citation: Machine learning predicts behaviour of stainless alloy astatine the microstructural level (2021, August 24) retrieved 24 August 2021 from https://techxplore.com/news/2021-08-machine-behavior-stainless-steel-microstructural.html
This papers is taxable to copyright. Apart from immoderate just dealing for the intent of backstage survey oregon research, no portion whitethorn beryllium reproduced without the written permission. The contented is provided for accusation purposes only.