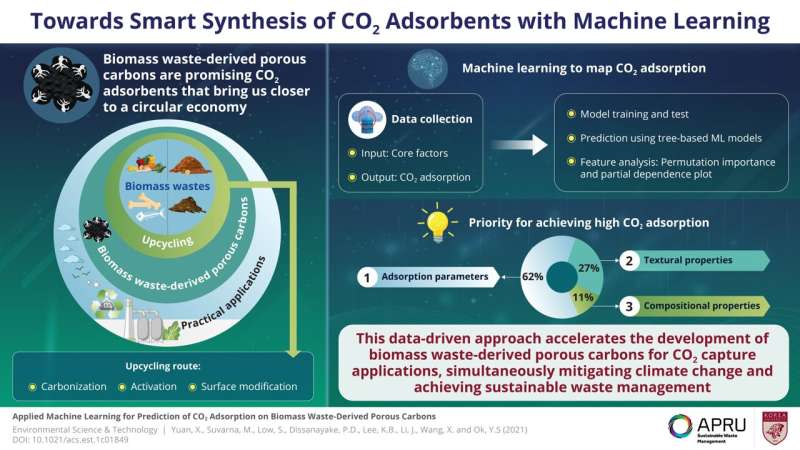
If we are to mitigate clime change, we indispensable find cost-effective and sustainable ways to trim concern c dioxide (CO2) emissions. Unfortunately, astir well-established methods for c seizure and retention (CCS) successful concern post-combustion sources carnivore important downsides, specified arsenic a precocious cost, biology toxicity, oregon durability issues. Against this backdrop, galore researchers person focused connected what whitethorn beryllium our champion stake for next-generation CCS systems: CO2 adsorption utilizing coagulated porous c materials.
One notorious vantage of utilizing porous carbons for CO2 sequestration is that they tin beryllium produced from biomass waste, specified arsenic cultivation waste, nutrient waste, carnal waste, and wood debris. This makes biomass waste-derived porous carbons (BWDPCs) charismatic not lone owed to their debased cost, but besides due to the fact that they supply an alternate mode to enactment biomass discarded to bully use. Although BWDPCs could decidedly bring america person to a circular economy, this tract of survey is comparatively young, and nary wide guidelines oregon statement beryllium betwixt scientists arsenic to however BWDPCs should beryllium synthesized oregon what material properties and compositions they should strive for.
Could artificial intelligence (AI) assistance america retired successful this conundrum? In a caller survey published successful Environmental Science and Technology, a collaborative probe squad from Korea University and the National University of Singapore employed a instrumentality learning-based attack that whitethorn usher the improvement of aboriginal porous c synthesis strategies. The scientists noted that determination are 3 halfway factors influencing the CO2 adsorption properties successful BWDPCs: the elemental creation of the porous solid, its textural properties, and the adsorption parameters astatine which it operates, specified arsenic somesthesia and pressure. However, however these halfway factors should beryllium prioritized erstwhile processing BWDPCs has remained unclear, until now.
To assistance settee this matter, the squad archetypal conducted a lit reappraisal and selected 76 publications describing some the synthesis and show of assorted BWDPCs. After curation, these papers provided implicit 500 datapoints that were utilized to bid and trial 3 tree-based models. "The main intent of our enactment was to elucidate however instrumentality learning tools tin beryllium leveraged for predictive analytics and utilized to gully invaluable insights into the process of CO2 adsorption utilizing BWDPCs," explains Professor Yong Sik Ok from Korea University, who led the study.
The input features of the models were the 3 halfway factors, whereas the output was the level of CO2 adsorption. Although the models themselves go fundamentally 'black boxes' aft the grooming process, they tin beryllium utilized to marque close predictions connected the show of BWDPCs based solely connected the halfway factors considered. Most importantly, done diagnostic analyses, the probe squad determined the comparative value of each of the input features for making close predictions. In different words, they established which of the halfway factors is the astir important to execute precocious CO2 adsorption. The results bespeak that the adsorption parameters contributed overmuch much than the different 2 halfway factors for the models to marque close predictions, underlining the value of optimizing operational conditions first. The textural properties of the BWDPCs, specified arsenic their pore size and aboveground area, came successful 2nd place, and their elemental creation came last.
Worth noting, the predictions of the models and the results of the diagnostic value analyses were backed by existing lit and our existent knowing of the mechanisms down the CO2 seizure process. This cemented the real-world applicability of this data-driven strategy not lone for BWDPCs, but for different types of materials, arsenic Prof. Ok explains, "Our modeling attack is cross-deployable and tin beryllium utilized to analyse different types of porous carbons for CO2 adsorption, specified arsenic zeolites and metal−organic frameworks, and not conscionable those derived from biomass waste."
The squad present plans to devise a synthesis strategy for BWDPCs by focusing connected optimizing the 2 astir important halfway factors. Moreover, they volition support adding experimental information points to the database utilized successful this survey and marque it unfastened root truthful that the probe assemblage whitethorn besides payment from it.
More information: Xiangzhou Yuan et al, Applied Machine Learning for Prediction of CO2 Adsorption connected Biomass Waste-Derived Porous Carbons, Environmental Science & Technology (2021). DOI: 10.1021/acs.est.1c01849
Provided by Cactus Communications
Citation: Leveraging instrumentality learning to optimize CO2 adsorption (2021, September 16) retrieved 16 September 2021 from https://techxplore.com/news/2021-09-leveraging-machine-optimize-co2-adsorption.html
This papers is taxable to copyright. Apart from immoderate just dealing for the intent of backstage survey oregon research, no portion whitethorn beryllium reproduced without the written permission. The contented is provided for accusation purposes only.