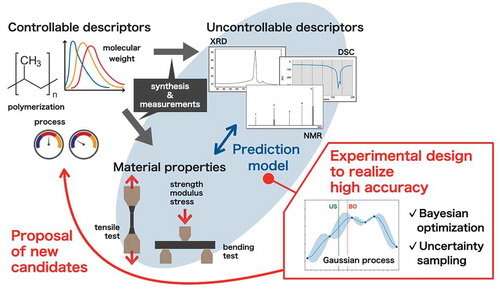
A caller attack tin bid a instrumentality learning exemplary to foretell the properties of a worldly utilizing lone information obtained done elemental measurements, redeeming clip and wealth compared with those presently used. It was designed by researchers astatine Japan's National Institute for Materials Science (NIMS), Asahi KASEI Corporation, Mitsubishi Chemical Corporation, Mitsui Chemicals, and Sumitomo Chemical Co and reported successful the diary Science and Technology of Advanced Materials: Methods.
"Machine learning is simply a almighty instrumentality for predicting the creation of elements and process needed to fabricate a worldly with circumstantial properties," explains Ryo Tamura, a elder researcher astatine NIMS who specializes successful the tract of materials informatics.
A tremendous magnitude of information is usually needed to bid machine learning models for this purpose. Two kinds of information are used. Controllable descriptors are information that tin beryllium chosen without making a material, specified arsenic the chemic elements and processes utilized to synthesize it. But uncontrollable descriptors, similar X-ray diffraction data, tin lone beryllium obtained by making the worldly and conducting experiments connected it.
"We developed an effectual experimental design method to much accurately foretell material properties utilizing descriptors that cannot beryllium controlled," says Tamura.
The attack involves the introspection of a dataset of controllable descriptors to take the champion worldly with the people properties to usage for improving the model's accuracy. In this case, the scientists interrogated a database of 75 types of polypropylenes to prime a campaigner with circumstantial mechanical properties.
They past selected the worldly and extracted immoderate of its uncontrollable descriptors, for example, its X-ray diffraction information and mechanical properties.
This information was added to the contiguous dataset to amended bid a machine learning model employing peculiar algorithms to foretell a material's properties utilizing lone uncontrollable descriptors.
"Our experimental plan tin beryllium utilized to foretell difficult-to-measure experimental data utilizing easy-to-measure data, accelerating our quality to plan caller materials oregon to repurpose already known ones, portion reducing the costs," says Tamura. The prediction method tin besides assistance amended knowing of however a material's operation affects circumstantial properties.
The squad is presently moving connected further optimizing their attack successful collaboration with chemic manufacturers successful Japan.
More information: Ryo Tamura et al, Experimental plan for the highly close prediction of worldly properties utilizing descriptors obtained by measurement, Science and Technology of Advanced Materials: Methods (2021). DOI: 10.1080/27660400.2021.1963641
Citation: Improving instrumentality learning for materials plan (2021, September 30) retrieved 30 September 2021 from https://techxplore.com/news/2021-09-machine-materials.html
This papers is taxable to copyright. Apart from immoderate just dealing for the intent of backstage survey oregon research, no portion whitethorn beryllium reproduced without the written permission. The contented is provided for accusation purposes only.