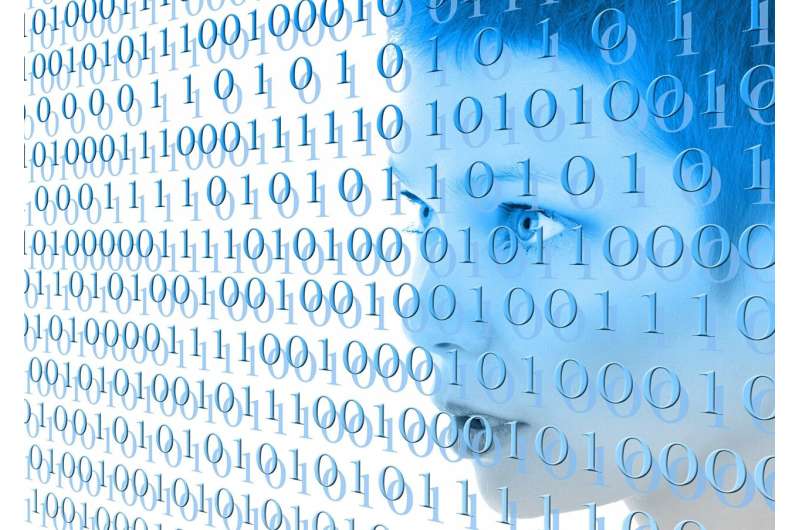
KAUST researchers person recovered a mode to importantly summation the velocity of training. Large instrumentality learning models tin beryllium trained importantly faster by observing however often zero results are produced successful distributed instrumentality learning that usage ample grooming datasets.
AI models make their "intelligence" by being trained connected datasets that person been labeled to archer the model however to differentiate betwixt antithetic inputs and past respond accordingly. The much labeled information that goes in, the amended the exemplary becomes astatine performing immoderate task it has been assigned to do. For analyzable heavy learning applications, specified arsenic self-driving vehicles, this requires tremendous input datasets and precise agelong training times, adjacent erstwhile utilizing almighty and costly highly parallel supercomputing platforms.
During training, tiny learning tasks are assigned to tens oregon hundreds of computing nodes, which past stock their results implicit a communications network earlier moving the adjacent task. One of the biggest sources of computing overhead successful specified parallel computing tasks is really this connection among computing nodes astatine each exemplary step.
"Communication is simply a large show bottleneck successful distributed heavy learning," explains Jiawei Fei from the KAUST team. "Along with the fast-paced summation successful exemplary size, we besides spot an summation successful the proportionality of zero values that are produced during the learning process, which we telephone sparsity. Our thought was to exploit this sparsity to maximize effectual bandwidth usage by sending lone non-zero information blocks."
Building connected an earlier KAUST improvement called SwitchML, which optimized internode communications by moving businesslike aggregation codification connected the web switches that process information transfer, Fei, Marco Canini and their colleagues went a measurement further by identifying zero results and processing a mode to driblet transmission without interrupting the synchronization of the parallel computing process.
"Exactly however to exploit sparsity to accelerate distributed grooming is simply a challenging problem," says Fei. "All nodes request to process information blocks astatine the aforesaid determination successful a time slot, truthful we person to coordinate the nodes to guarantee that lone information blocks successful the aforesaid determination are aggregated. To flooded this, we created an aggregator process to coordinate the workers, instructing them connected which artifact to nonstop next."
The squad demonstrated their OmniReduce strategy connected a testbed consisting of an array of graphics processing units (GPU) and achieved an eight-fold speed-up for emblematic deep learning tasks.
"We are present adapting OmniReduce to tally connected programmable switches utilizing in-network computation to further amended performance," Fei says.
More information: Jiawei Fei et al, Efficient sparse corporate connection and its exertion to accelerate distributed heavy learning, Proceedings of the 2021 ACM SIGCOMM 2021 Conference (2021). DOI: 10.1145/3452296.3472904
Citation: Improve instrumentality learning show by dropping the zeros (2021, August 23) retrieved 23 August 2021 from https://techxplore.com/news/2021-08-machine-zeros.html
This papers is taxable to copyright. Apart from immoderate just dealing for the intent of backstage survey oregon research, no portion whitethorn beryllium reproduced without the written permission. The contented is provided for accusation purposes only.