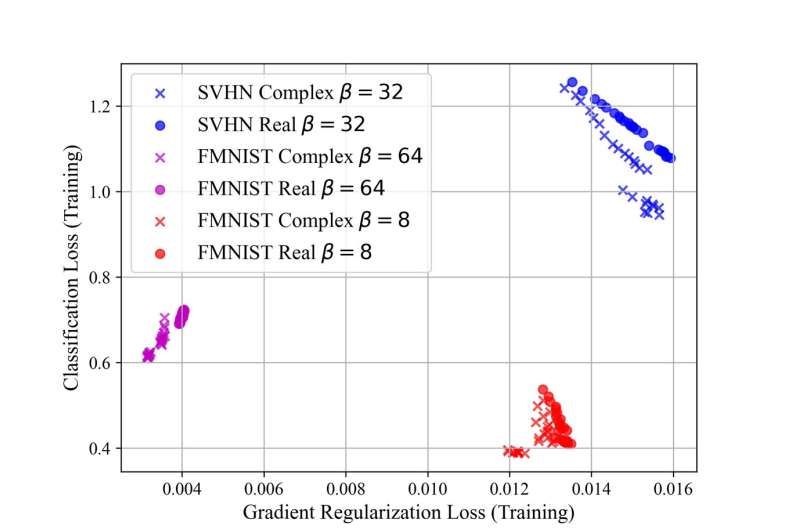
Computer engineers astatine Duke University person demonstrated that utilizing analyzable numbers—numbers with some existent and imaginary components—can play an integral portion successful securing artificial quality algorithms against malicious attacks that effort to fool object-identifying bundle by subtly altering the images. By including conscionable 2 complex-valued layers among hundreds if not thousands of grooming iterations, the method tin amended show against specified attacks without sacrificing immoderate efficiency.
The probe was presented astatine the Proceedings of the 38th International Conference connected Machine Learning.
"We're already seeing instrumentality learning algorithms being enactment to usage successful the real world that are making existent decisions successful areas similar conveyance autonomy and facial recognition," said Eric Yeats, a doctoral pupil moving successful the laboratory of Helen Li, the Clare Boothe Luce Professor of Electrical and Computer Engineering astatine Duke. "We request to deliberation of ways to guarantee that these algorithms are reliable to marque definite they can't origin immoderate problems oregon wounded anyone."
One mode that instrumentality learning algorithms built to place objects and images tin beryllium fooled is done adversarial attacks. This fundamentally entails modifying the representation successful a mode that breaks the AI's decision-making process. It tin beryllium arsenic elemental arsenic adding stickers to a stop sign oregon arsenic blase arsenic adding a cautiously crafted furniture of static to an representation that alters it successful ways undetectable to the quality eye.
The crushed these tiny perturbations tin origin specified ample problems stems from however instrumentality learning algorithms are trained. One modular method called gradient descent compares the decisions it arrives astatine to the correct answers, attempts to tweak its interior workings to hole the errors, and repeats the process implicit and implicit again until it is nary longer improving.
One mode to visualize this is to ideate a boulder rolling done a vale of hills and mountains. With each machine learning iteration, the algorithm's moving parameters (boulder) rolls further into the valley. When it starts to rotation up a caller hill, the algorithm changes its people to support it rolling downward. Eventually the boulder settles successful the champion reply (lowest spot) around.
A challenging facet of this attack is that the vale the boulder is rolling done is particularly rugged terrain—think the Himalayas alternatively of the Appalachians. One tiny nudge successful the incorrect absorption tin nonstop the boulder plummeting toward a precise antithetic outcome. This is wherefore hardly noticeable static tin marque an representation classifier spot a gibbon alternatively of a panda.
To support their algorithms connected track, machine scientists tin bid their algorithms with a method called gradient regularization. This causes the boulder to take paths that aren't arsenic steep. While the causes the boulder to instrumentality a different—and longer—path to its last resting spot, it besides makes definite the boulder rolls mildly down the close vale alternatively of being pushed disconnected a adjacent ravine.
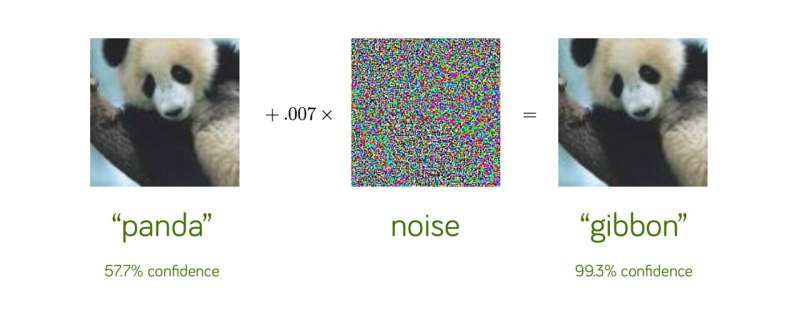
"Gradient regularization throws retired immoderate solution that passes a ample gradient backmost done the neural network," Yeats said. "This reduces the fig of solutions that it could get at, which besides tends to alteration however good the algorithm really arrives astatine the close answer. That's wherever analyzable values tin help. Given the aforesaid parameters and mathematics operations, utilizing analyzable values is much susceptible of resisting this alteration successful performance."
Chances are astir of america haven't thought about—or adjacent heard the words—imaginary numbers since astir 8th grade. And their instauration was apt accompanied by groans followed by a chorus of, "What americium I ever going to usage this for?" But imaginary numbers are extraordinarily utile for describing sinusoidal waves, which hap to look a batch similar a vale of hills and mountains.
When the neural web is being trained connected a acceptable of images, utilizing analyzable numbers with imaginary components gives it added flexibility successful however it adjusts its interior parameters to get astatine a solution. Rather than lone being capable to multiply and accumulate changes, it tin offset the signifier of the waves it's adding together, allowing them to either amplify oregon cancel 1 different out. The effect is that this once-rugged vale is smoothed retired to locally flatter surfaces with aggregate tiers that let for tons of elevation alteration successful different areas.
"The complex-valued neural networks person the imaginable for a much 'terraced' oregon 'plateaued' scenery to explore," Yeates said. "And elevation alteration lets the neural network conceive much analyzable things, which means it tin place much objects with much precision."
That added capableness allows gradient regularization neural networks utilizing analyzable numbers to find solutions conscionable arsenic accelerated arsenic those trained without the other security. In his research, Yeats shows that representation classifiers aimed astatine recognizing location numbers from Google Maps and antithetic covering items trained connected his attack are much unafraid than modular methods portion performing astatine the aforesaid level.
"This is inactive an unfastened and challenging problem," Yeats said. "So researchers are doing what they tin to bash a small spot amended present and there."
More information: "Improving Gradient Regularization utilizing Complex-Valued Neural Networks." Eric Yeats, Yiran Chen, Hai Li. Proceedings of the 38th International Conference connected Machine Learning, PMLR 139, 2021.
Citation: Imaginary numbers support AI from precise existent threats (2021, September 1) retrieved 1 September 2021 from https://techxplore.com/news/2021-09-imaginary-ai-real-threats.html
This papers is taxable to copyright. Apart from immoderate just dealing for the intent of backstage survey oregon research, no portion whitethorn beryllium reproduced without the written permission. The contented is provided for accusation purposes only.