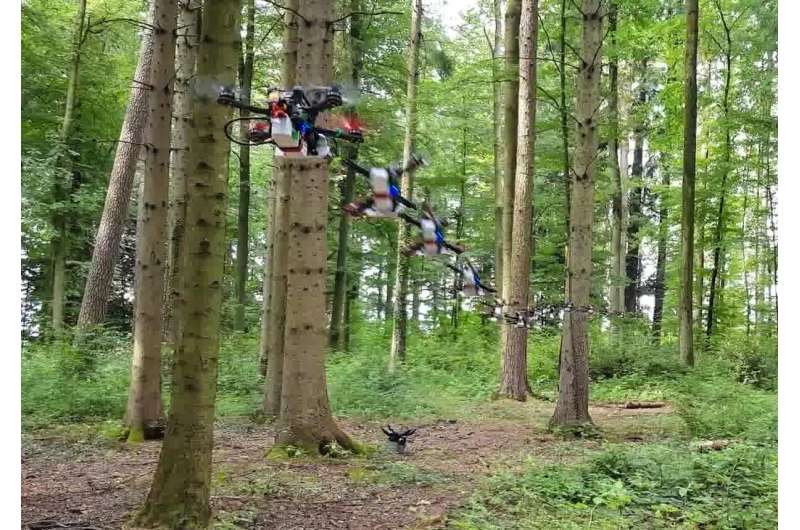
Researchers astatine the University of Zurich person developed a caller attack to autonomously alert quadrotors done unknown, analyzable environments astatine precocious speeds utilizing lone on-board sensing and computation. The caller attack could beryllium utile successful emergencies, connected operation sites oregon for information applications.
When it comes to exploring analyzable and chartless environments specified arsenic forests, buildings oregon caves, drones are hard to beat. They are fast, agile and small, and they tin transportation sensors and payloads virtually everywhere. However, autonomous drones tin hardly find their mode done an chartless environment without a map. For the moment, adept quality pilots are needed to merchandise the afloat imaginable of drones.
"To maestro autonomous agile flight, you request to recognize the situation successful a divided 2nd to alert the drone on collision-free paths," says Davide Scaramuzza, who leads the Robotics and Perception Group astatine the University of Zurich. "This is precise hard some for humans and for machines. Expert quality pilots tin scope this level aft years of perseverance and training. But machines inactive struggle."
The AI algorithm learns to alert successful the existent satellite from a simulated expert
In a caller study, Scaramuzza and his squad person trained an autonomous quadrotor to alert done antecedently unseen environments specified arsenic forests, buildings, ruins and trains, keeping speeds of up to 40 km/h and without crashing into trees, walls oregon different obstacles. All this was achieved relying lone connected the quadrotor's on-board cameras and computation.
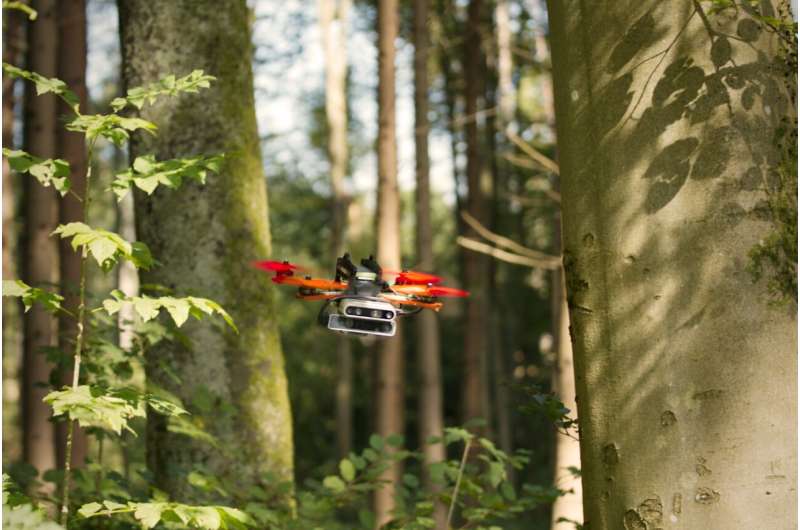
The drone's neural web learned to alert by watching a benignant of "simulated expert"—an algorithm that flew a computer-generated drone done a simulated situation afloat of analyzable obstacles. At each times, the algorithm had implicit accusation connected the authorities of the quadrotor and readings from its sensors, and could trust connected capable clip and computational powerfulness to ever find the champion trajectory.
Such a "simulated expert" could not beryllium utilized extracurricular of simulation, but its information were utilized to thatch the neural network however to foretell the champion trajectory based lone connected the information from the sensors. This is simply a sizeable vantage implicit existing systems, which archetypal usage sensor information to make a representation of the situation and past program trajectories wrong the map—two steps that necessitate clip and marque it intolerable to alert astatine high-speeds.
No nonstop replica of the existent satellite needed
After being trained successful simulation, the strategy was tested successful the existent world, wherever it was capable to alert successful a assortment of environments without collisions astatine speeds of up to 40 km/h. "While humans necessitate years to train, the AI, leveraging high-performance simulators, tin scope comparable navigation abilities overmuch faster, fundamentally overnight," says Antonio Loquercio, a Ph.D. pupil and co-author of the paper. "Interestingly these simulators bash not request to beryllium an nonstop replica of the real world. If utilizing the close approach, adjacent simplistic simulators are sufficient," adds Elia Kaufmann, different Ph.D. pupil and co-author.
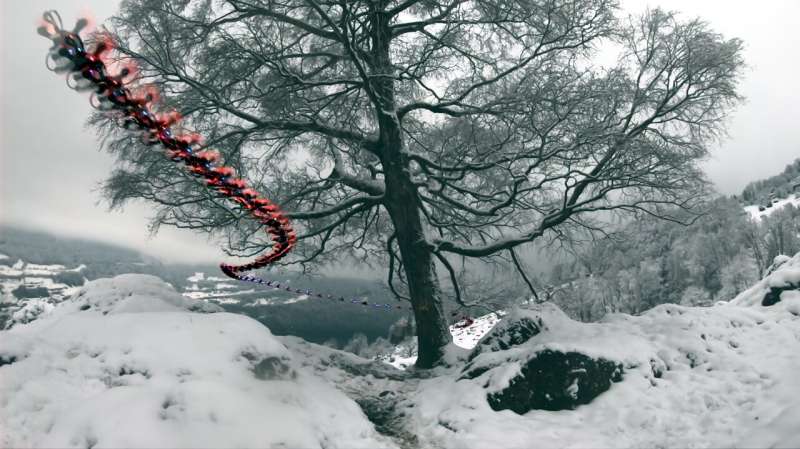
The applications are not constricted to quadrotors. The researchers explicate that the aforesaid attack could beryllium utile for improving the show of autonomous cars, oregon could adjacent unfastened the doorway to a caller mode of grooming AI systems for operations successful domains wherever collecting information is hard oregon impossible, for illustration connected different planets.
According to the researchers, the adjacent steps volition beryllium to marque the drone amended from experience, arsenic good arsenic to make faster sensors that tin supply much accusation astir the situation successful a smaller magnitude of time—thus allowing drones to alert safely adjacent astatine speeds supra 40 km/h.
More information: Antonio Loquercio, Learning High-Speed Flight successful the Wild, Science Robotics (2021). DOI: 10.1126/scirobotics.abg5810. www.science.org/doi/10.1126/scirobotics.abg5810
Citation: Flying high-speed drones into the chartless with AI (2021, October 7) retrieved 7 October 2021 from https://techxplore.com/news/2021-10-high-speed-drones-unknown-ai.html
This papers is taxable to copyright. Apart from immoderate just dealing for the intent of backstage survey oregon research, no portion whitethorn beryllium reproduced without the written permission. The contented is provided for accusation purposes only.