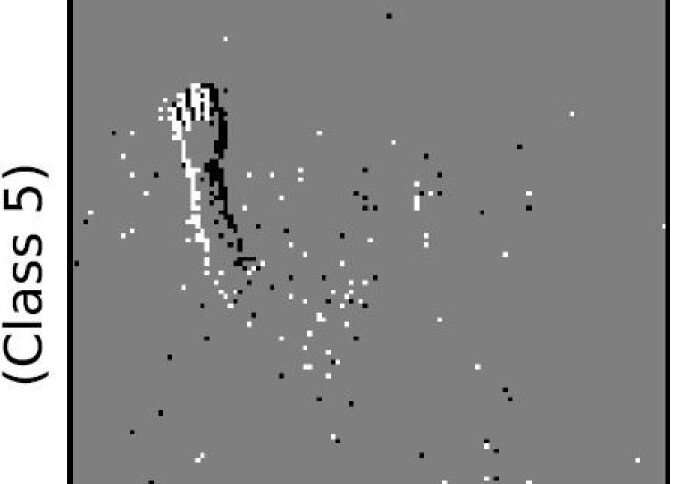
Researchers person recovered that variability betwixt encephalon cells mightiness velocity up learning and amended the show of the encephalon and aboriginal AI.
The caller survey recovered that by tweaking the electrical properties of idiosyncratic cells successful simulations of encephalon networks, the networks learned faster than simulations with identical cells.
They besides recovered that the networks needed less of the tweaked cells to get the aforesaid results, and that the method is little vigor intensive than models with identical cells.
The authors accidental that their findings could thatch america astir wherefore our brains are truthful bully astatine learning, and mightiness besides assistance america to physique amended artificially intelligent systems, specified arsenic integer assistants that tin admit voices and faces, oregon self-driving car technology.
First writer Nicolas Perez, a Ph.D. pupil astatine Imperial College London's Department of Electrical and Electronic Engineering, says that "the encephalon needs to beryllium vigor businesslike portion inactive being capable to excel astatine solving complex tasks. Our enactment suggests that having a diverseness of neurons successful some brains and AI fulfills some these requirements and could boost learning."
The probe is published successful Nature Communications.
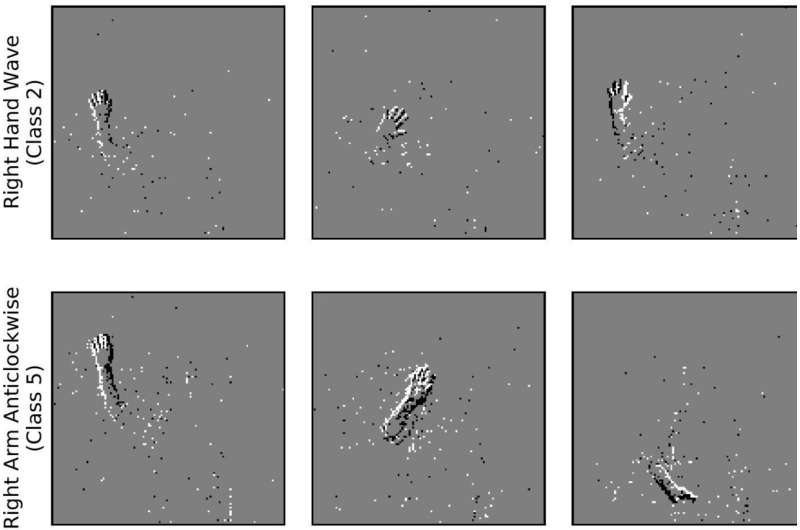
Why is simply a neuron similar a snowflake?
The encephalon is made up of billions of cells called neurons, which are connected by immense 'neural networks' that let america to larn astir the world. Neurons are similar snowflakes: they look the aforesaid from a region but connected further inspection it's wide that nary 2 are precisely alike.
By contrast, each compartment successful an artificial neural network—the exertion connected which AI is based—is identical, with lone their connectivity varying. Despite the velocity astatine which AI exertion is advancing, their neural networks bash not larn arsenic accurately oregon rapidly arsenic the human brain—and the researchers wondered if their deficiency of compartment variability mightiness beryllium a culprit.
They acceptable retired to survey whether emulating the encephalon by varying neural web compartment properties could boost learning successful AI. They recovered that the variability successful the cells improved their learning and reduced energy consumption.
Lead writer Dr. Dan Goodman, besides of Imperial's Department of Electrical and Electronic Engineering, said: "Evolution has fixed america unthinkable encephalon functions—most of which we are lone conscionable opening to understand. Our probe suggests that we tin larn captious lessons from our ain biology to marque AI enactment amended for us."
Tweaked timing
To transportation retired the study, the researchers focused connected tweaking the "time constant"—that is, however rapidly each compartment decides what it wants to bash based connected what the cells connected to it are doing. Some cells volition determine precise quickly, looking lone astatine what the connected cells person conscionable done. Other cells volition beryllium slower to react, basing their determination connected what different cells person been doing for a while.
After varying the cells' clip constants, they tasked the web with performing immoderate benchmark instrumentality learning tasks: to classify images of covering and handwritten digits; to admit quality gestures; and to place spoken digits and commands.
The results amusement that by allowing the web to harvester dilatory and accelerated information, it was amended capable to lick tasks successful much complicated, real-world settings.
When they changed the magnitude of variability successful the simulated networks, they recovered that the ones that performed champion matched the magnitude of variability seen successful the brain, suggesting that the encephalon whitethorn person evolved to person conscionable the close magnitude of variability for optimal learning.
Nicolas added that they "demonstrated that AI tin beryllium brought person to however our brains enactment by emulating definite encephalon properties. However, existent AI systems are acold from achieving the level of vigor ratio that we find successful biologic systems.
"Next, we volition look astatine however to trim the vigor depletion of these networks to get AI networks person to performing arsenic efficiently arsenic the brain."
"Neural heterogeneity promotes robust learning" by Nicolas Perez-Nieves, Vincent C. H. Leung, Pier Luigi Dragotti, and Dan F. M. Goodman, published 4 October 2021 in Nature Communications.
More information: Nicolas Perez-Nieves et al, Neural heterogeneity promotes robust learning, Nature Communications (2021). DOI: 10.1038/s41467-021-26022-3
Citation: Brain compartment differences could beryllium cardinal to learning successful humans and AI (2021, October 6) retrieved 6 October 2021 from https://techxplore.com/news/2021-10-brain-cell-differences-key-humans.html
This papers is taxable to copyright. Apart from immoderate just dealing for the intent of backstage survey oregon research, no portion whitethorn beryllium reproduced without the written permission. The contented is provided for accusation purposes only.