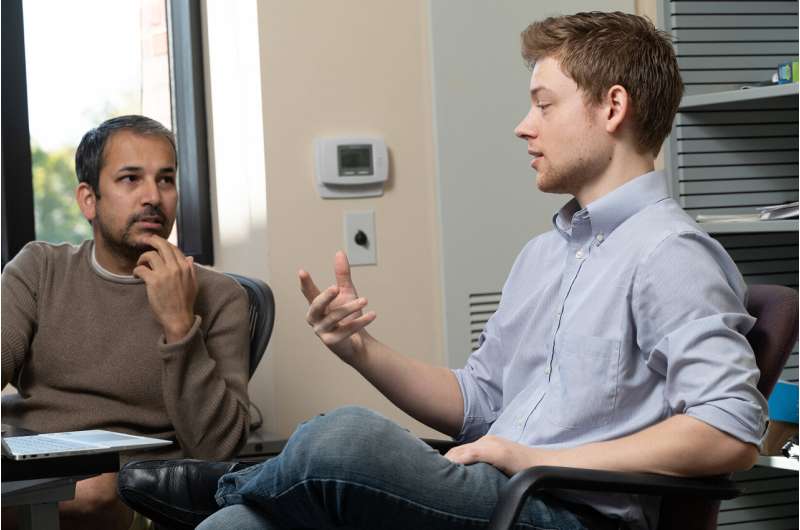
Rice University machine scientists person discovered an inexpensive mode for tech companies to instrumentality a rigorous signifier of idiosyncratic information privateness erstwhile utilizing oregon sharing ample databases for instrumentality learning.
"There are galore cases wherever machine learning could payment nine if information privateness could beryllium ensured," said Anshumali Shrivastava, an subordinate prof of machine subject astatine Rice. "There's immense imaginable for improving aesculapian treatments oregon uncovering patterns of discrimination, for example, if we could bid instrumentality learning systems to hunt for patterns successful ample databases of aesculapian oregon fiscal records. Today, that's fundamentally intolerable due to the fact that information privateness methods bash not scale."
Shrivastava and Rice postgraduate pupil Ben Coleman anticipation to alteration that with a caller method they'll contiguous this week astatine CCS 2021, the Association for Computing Machinery's yearly flagship league connected machine and communications security. Using a method called locality delicate hashing, Shirvastava and Coleman recovered they could make a tiny summary of an tremendous database of delicate records. Dubbed RACE, their method draws its sanction from these summaries, oregon "repeated array of number estimators" sketches.
Coleman said RACE sketches are some harmless to marque publically disposable and utile for algorithms that usage kernel sums, 1 of the basal gathering blocks of instrumentality learning, and for machine-learning programs that execute communal tasks similar classification, ranking and regression analysis. He said RACE could let companies to some reap the benefits of large-scale, distributed instrumentality learning and uphold a rigorous signifier of data privacy called differential privacy.
Differential privacy, which is utilized by much than 1 tech giant, is based connected the thought of adding random sound to obscure idiosyncratic information.
"There are elegant and almighty techniques to conscionable differential privateness standards today, but nary of them scale," Coleman said. "The computational overhead and the representation requirements turn exponentially arsenic information becomes much dimensional."
Data is progressively high-dimensional, meaning it contains some galore observations and galore idiosyncratic features astir each observation.
RACE sketching scales for high-dimensional data, helium said. The sketches are tiny and the computational and representation requirements for constructing them are besides casual to distribute.
"Engineers contiguous indispensable either sacrifice their fund oregon the privateness of their users if they privation to usage kernel sums," Shrivastava said. "RACE changes the economics of releasing high-dimensional accusation with differential privacy. It's simple, accelerated and 100 times little costly to tally than existing methods."
This is the latest innovation from Shrivasta and his students, who person developed galore algorithmic strategies to marque instrumentality learning and information subject faster and much scalable. They and their collaborators have: recovered a much businesslike mode for societal media companies to support misinformation from spreading online, discovered however to bid large-scale heavy learning systems up to 10 times faster for "extreme classification" problems, recovered a mode to much accurately and efficiently estimate the fig of identified victims killed successful the Syrian civilian war, showed it's possible to bid deep neural networks arsenic overmuch arsenic 15 times faster connected wide intent CPUs (central processing units) than GPUs (graphics processing units), and slashed the magnitude of clip required for searching ample metagenomic databases.
More information: Benjamin Coleman et al, A One-Pass Private Sketch for Most Machine Learning Tasks, arXiv:2006.09352 [cs.DS], arxiv.org/abs/2006.09352
Citation: Big information privateness for instrumentality learning conscionable got 100 times cheaper (2021, November 16) retrieved 16 November 2021 from https://techxplore.com/news/2021-11-big-privacy-machine-cheaper.html
This papers is taxable to copyright. Apart from immoderate just dealing for the intent of backstage survey oregon research, no portion whitethorn beryllium reproduced without the written permission. The contented is provided for accusation purposes only.