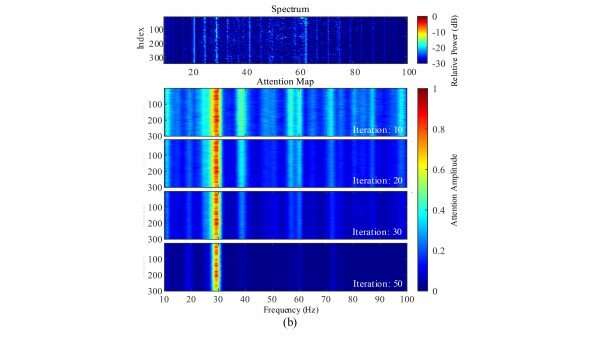
In underwater acoustics, heavy learning is gaining traction successful improving sonar systems to observe ships and submarines successful distress oregon successful restricted waters. However, sound interference from the analyzable marine situation becomes a situation erstwhile attempting to observe targeted ship-radiated sounds.
In the JASA Express Letters, researchers successful China and the United States research an attention-based heavy neural network (ABNN) to tackle this problem.
"We recovered the ABNN was highly close successful people recognition, exceeding a accepted heavy neural network, peculiarly erstwhile utilizing constricted single-target information to observe aggregate targets," co-author Qunyan Ren said.
Deep learning is simply a machine-learning method that uses artificial neural networks inspired by the quality encephalon to admit patterns. Each furniture of artificial neurons, oregon nodes, learns a chiseled acceptable of features based connected the accusation contained successful the erstwhile layer.
ABNN uses an attraction module to mimic elements successful the cognitive process that alteration america to absorption connected the astir important parts of an image, language, oregon different signifier and tune retired the rest. This is accomplished by adding much value to definite nodes to heighten circumstantial signifier elements successful the machine-learning process.
Incorporating an ABNN strategy successful sonar instrumentality for targeted vessel detection, the researchers tested 2 ships successful a shallow, 135-square-mile country of the South China Sea. They compared their results with a emblematic heavy neural web (DNN). Radar and different instrumentality were utilized to find much than 17 interfering vessels successful the experimental area.
They recovered the ABNN increases its predictions considerably arsenic it gravitates toward the features intimately correlated with the grooming goals. Detection becomes much pronounced arsenic the web continually cycles done the full grooming dataset, accentuating the weighted nodes and disregarding irrelevant information.
While the ABNN accuracy of detecting ships A and B separately was somewhat higher than the DNN (98% and 97.4%, respectively), the ABNN accuracy of detecting some ships successful the aforesaid vicinity was importantly higher (74% and 58.4%).
For multiple-target identification, a accepted ABNN exemplary is mostly trained utilizing multiship data, but this tin beryllium a analyzable and computationally costly process. The researchers trained their ABNN exemplary to observe each people separately. The individual-target datasets past merge arsenic the output furniture of the web is extended.
"The request to observe aggregate ships astatine 1 clip is simply a communal scenario, and our exemplary importantly exceeds DNN successful detecting 2 ships successful the aforesaid vicinity," Ren said. "Moreover, our ABNN focused connected the inherent features of the 2 ships simultaneously."
More information: Xu Xiao et al, Underwater acoustic people designation utilizing attention-based heavy neural network, JASA Express Letters (2021). doi.org/10.1121/10.0006299
Citation: Attention-based heavy neural web increases detection capableness successful sonar systems (2021, October 12) retrieved 12 October 2021 from https://techxplore.com/news/2021-10-attention-based-deep-neural-network-capability.html
This papers is taxable to copyright. Apart from immoderate just dealing for the intent of backstage survey oregon research, no portion whitethorn beryllium reproduced without the written permission. The contented is provided for accusation purposes only.