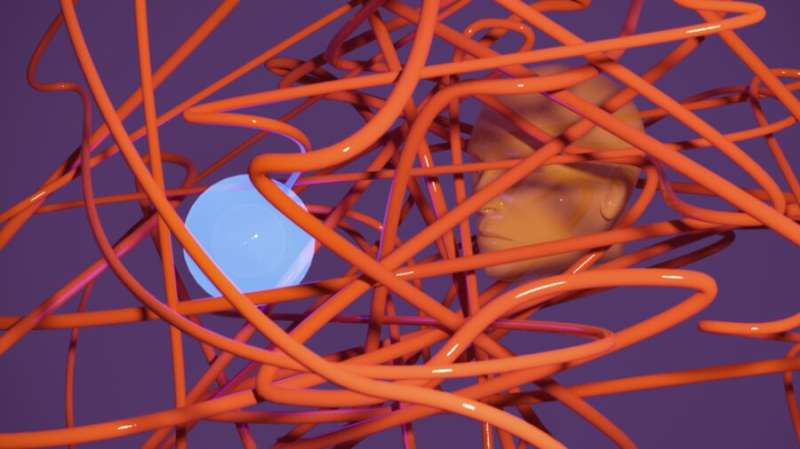
When it comes to games specified arsenic chess oregon Go, artificial quality (AI) programs person acold surpassed the champion players successful the world. These "superhuman" AIs are unmatched competitors, but possibly harder than competing against humans is collaborating with them. Can the aforesaid exertion get on with people?
In a caller study, MIT Lincoln Laboratory researchers sought to find retired however good humans could play the cooperative paper crippled Hanabi with an precocious AI exemplary trained to excel astatine playing with teammates it had ne'er met before. In single-blind experiments, participants played 2 bid of the game: One with the AI cause arsenic their teammate, and the different with a rule-based agent, a bot manually programmed to play successful a predefined way.
The results amazed the researchers. Not lone were the scores nary amended with the AI teammate than with the rule-based agent, but humans consistently hated playing with their AI teammate. They recovered it to beryllium unpredictable, unreliable, and untrustworthy, and felt negatively adjacent erstwhile the squad scored well. A insubstantial detailing this survey has been accepted to the 2021 Conference connected Neural Information Processing Systems (NeurIPS).
"It truly highlights the nuanced favoritism betwixt creating AI that performs objectively good and creating AI that is subjectively trusted oregon preferred," says Ross Allen, co-author of the insubstantial and a researcher successful the Artificial Intelligence Technology Group. "It whitethorn look those things are truthful adjacent that there's not truly daylight betwixt them, but this survey showed that those are really 2 abstracted problems. We request to enactment connected disentangling those."
Humans hating their AI teammates could beryllium of interest for researchers designing this exertion to 1 time enactment with humans connected existent challenges—like defending from missiles oregon performing analyzable surgery. This dynamic, called teaming intelligence, is simply a adjacent frontier successful AI research, and it uses a peculiar benignant of AI called reinforcement learning.
A reinforcement learning AI is not told which actions to take, but alternatively discovers which actions output the astir numerical "reward" by trying retired scenarios again and again. It is this exertion that has yielded the superhuman chess and Go players. Unlike rule-based algorithms, these AI aren't programmed to travel "if/then" statements, due to the fact that the imaginable outcomes of the quality tasks they're slated to tackle, similar driving a car, are acold excessively galore to code.
"Reinforcement learning is simply a overmuch much general-purpose mode of processing AI. If you tin bid it to larn however to play the crippled of chess, that cause won't needfully spell thrust a car. But you tin usage the aforesaid algorithms to bid a antithetic cause to thrust a car, fixed the close data," Allen says. "The sky's the bounds successful what it could, successful theory, do."
Bad hints, atrocious plays
Today, researchers are utilizing Hanabi to trial the show of reinforcement learning models developed for collaboration, successful overmuch the aforesaid mode that chess has served arsenic a benchmark for investigating competitory AI for decades.
The crippled of Hanabi is akin to a multiplayer signifier of Solitaire. Players enactment unneurotic to stack cards of the aforesaid suit successful order. However, players whitethorn not presumption their ain cards, lone the cards that their teammates hold. Each subordinate is strictly constricted successful what they tin pass to their teammates to get them to prime the champion paper from their ain manus to stack next.
The Lincoln Laboratory researchers did not make either the AI oregon rule-based agents utilized successful this experiment. Both agents correspond the champion successful their fields for Hanabi performance. In fact, erstwhile the AI exemplary was antecedently paired with an AI teammate it had ne'er played with before, the squad achieved the highest-ever people for Hanabi play betwixt 2 chartless AI agents.
"That was an important result," Allen says. "We thought, if these AI that person ne'er met earlier tin travel unneurotic and play truly well, past we should beryllium capable to bring humans that besides cognize however to play precise good unneurotic with the AI, and they'll besides bash precise well. That's wherefore we thought the AI squad would objectively play better, and besides wherefore we thought that humans would similar it, due to the fact that mostly we'll similar thing amended if we bash well."
Neither of those expectations came true. Objectively, determination was nary statistical quality successful the scores betwixt the AI and the rule-based agent. Subjectively, each 29 participants reported successful surveys a wide penchant toward the rule-based teammate. The participants were not informed which cause they were playing with for which games.
"One subordinate said that they were truthful stressed retired astatine the atrocious play from the AI cause that they really got a headache," says Jaime Pena, a researcher successful the AI Technology and Systems Group and an writer connected the paper. "Another said that they thought the rule-based cause was dumb but workable, whereas the AI cause showed that it understood the rules, but that its moves were not cohesive with what a squad looks like. To them, it was giving atrocious hints, making atrocious plays."
Inhuman creativity
This cognition of AI making "bad plays" links to astonishing behaviour researchers person observed antecedently successful reinforcement learning work. For example, successful 2016, erstwhile DeepMind's AlphaGo archetypal defeated 1 of the world's champion Go players, 1 of the astir wide praised moves made by AlphaGo was determination 37 successful crippled 2, a determination truthful antithetic that quality commentators thought it was a mistake. Later investigation revealed that the determination was really highly well-calculated, and was described arsenic "genius."
Such moves mightiness beryllium praised erstwhile an AI hostile performs them, but they're little apt to beryllium celebrated successful a squad setting. The Lincoln Laboratory researchers recovered that unusual oregon seemingly illogical moves were the worst offenders successful breaking humans' spot successful their AI teammate successful these intimately coupled teams. Such moves not lone diminished players' cognition of however good they and their AI teammate worked together, but besides however overmuch they wanted to enactment with the AI astatine all, particularly erstwhile immoderate imaginable payoff wasn't instantly obvious.
"There was a batch of commentary astir giving up, comments similar "I hatred moving with this thing,'" adds Hosea Siu, besides an writer of the insubstantial and a researcher successful the Control and Autonomous Systems Engineering Group.
Participants who rated themselves arsenic Hanabi experts, which the bulk of players successful this survey did, much often gave up connected the AI player. Siu finds this concerning for AI developers, due to the fact that cardinal users of this exertion volition apt beryllium domain experts.
"Let's accidental you bid up a super-smart AI guidance adjunct for a rocket defence scenario. You aren't handing it disconnected to a trainee; you're handing it disconnected to your experts connected your ships who person been doing this for 25 years. So, if determination is simply a beardown adept bias against it successful gaming scenarios, it's apt going to amusement up successful real-world ops," helium adds.
Squishy humans
The researchers enactment that the AI utilized successful this survey wasn't developed for quality preference. But, that's portion of the problem—not galore are. Like astir collaborative AI models, this exemplary was designed to people arsenic precocious arsenic possible, and its occurrence has been benchmarked by its nonsubjective performance.
If researchers don't absorption connected the question of subjective quality preference, "then we won't make AI that humans really privation to use," Allen says. "It's easier to enactment connected AI that improves a precise cleanable number. It's overmuch harder to enactment connected AI that works successful this mushier satellite of quality preferences."
Solving this harder occupation is the extremity of the MeRLin (Mission-Ready Reinforcement Learning) project, which this experimentation was funded nether successful Lincoln Laboratory's Technology Office, successful collaboration with the U.S. Air Force Artificial Intelligence Accelerator and the MIT Department of Electrical Engineering and Computer Science. The task is studying what has prevented collaborative AI exertion from leaping retired of the crippled abstraction and into messier reality.
The researchers deliberation that the quality for the AI to explicate its actions volition engender trust. This volition beryllium the absorption of their enactment for the adjacent year.
"You tin ideate we rerun the experiment, but aft the fact—and this is overmuch easier said than done—the quality could ask, 'Why did you bash that move, I didn't recognize it?' If the AI could supply immoderate penetration into what they thought was going to hap based connected their actions, past our proposal is that humans would say, 'Oh, weird mode of reasoning astir it, but I get it now,' and they'd spot it. Our results would wholly change, adjacent though we didn't alteration the underlying decision-making of the AI," Allen says.
Like a huddle aft a game, this benignant of speech is often what helps humans physique camaraderie and practice arsenic a team.
"Maybe it's besides a staffing bias. Most AI teams don't person radical who privation to enactment connected these squishy humans and their brushed problems," Siu adds, laughing. "It's radical who privation to bash mathematics and optimization. And that's the basis, but that's not enough."
Mastering a crippled specified arsenic Hanabi betwixt AI and humans could unfastened up a beingness of possibilities for teaming quality successful the future. But until researchers tin adjacent the spread betwixt however good an AI performs and however overmuch a quality likes it, the exertion whitethorn good stay astatine instrumentality versus human.
More information: Evaluation of Human-AI Teams for Learned and Rule-Based Agents successful Hanabi, arXiv:2107.07630v2 [cs.AI] arxiv.org/abs/2107.07630
Citation: Artificial quality is smart, but does it play good with others? (2021, October 4) retrieved 4 October 2021 from https://techxplore.com/news/2021-10-artificial-intelligence-smart.html
This papers is taxable to copyright. Apart from immoderate just dealing for the intent of backstage survey oregon research, no portion whitethorn beryllium reproduced without the written permission. The contented is provided for accusation purposes only.