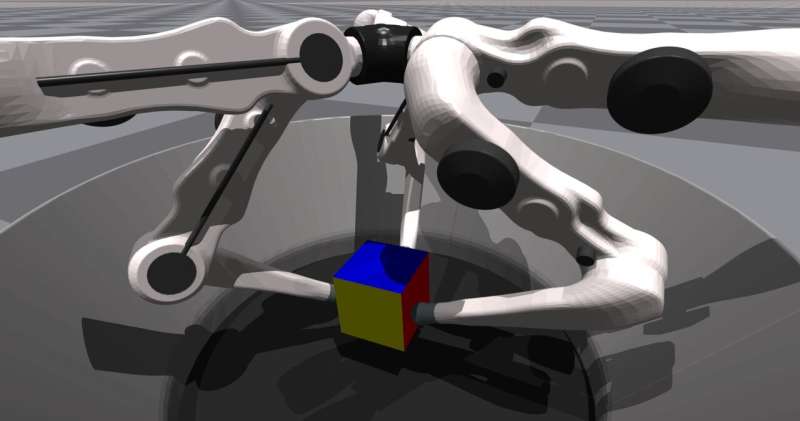
Last year, the Max Planck Institute for Intelligent Systems organized the Real Robot Challenge, a contention that challenged world labs to travel up with solutions to the occupation of repositioning and reorienting a cube utilizing a low-cost robotic hand. The teams participating successful the situation were asked to lick a bid of entity manipulation problems with varying trouble levels.
To tackle 1 of the problems posed by the Real Robot Challenge, researchers astatine University of Toronto's Vector Institute, ETH Zurich and MPI Tubingen developed a strategy that allows robots to get challenging dexterous manipulation skills, efficaciously transferring these skills from simulations to a existent robot. This system, presented successful a insubstantial pre-published connected arXiv, achieved a singular occurrence complaint of 83% successful allowing the distant TriFinger strategy projected by the situation organizers to implicit challenging tasks that progressive dexterous manipulation.
"Our nonsubjective was to usage learning-based methods to lick the occupation introduced successful past year's Real Robot Challenge successful a low-cost manner," Animesh Garg, 1 of the researchers who carried retired the study, told TechXplore. "We are peculiarly inspired by erstwhile enactment connected OpenAI's Dactyl system, which showed that it is imaginable to usage exemplary escaped Reinforcement Learning successful operation with Domain Randomization to lick analyzable manipulation tasks."
Essentially, Garg and his colleagues wanted to show that they could lick dexterous manipulation tasks utilizing a Trifinger robotic system, transferring results achieved successful simulations to the real world utilizing less resources than those employed successful erstwhile studies. To bash this, they trained a reinforcement learning cause successful simulations and created a deep learning technique that tin program aboriginal actions based connected a robot's observations.
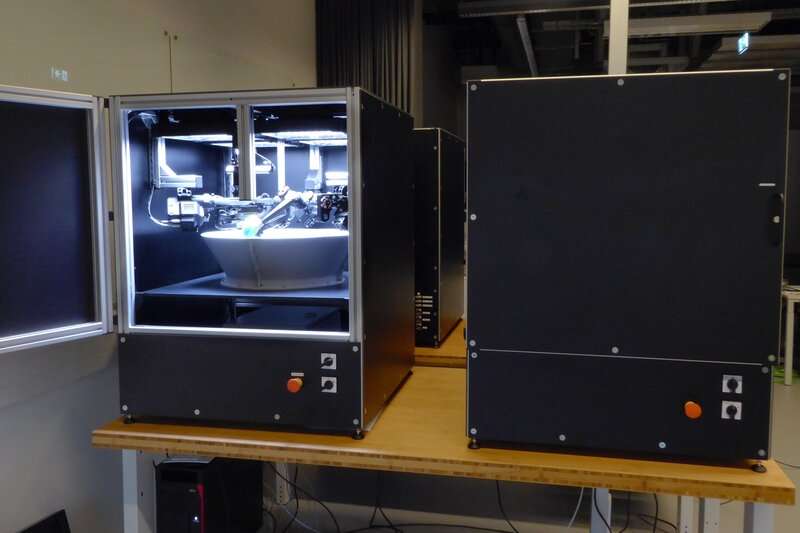
"The process we followed consists of 4 main steps: mounting up the situation successful physics simulation, choosing the close parameterization for a occupation specification, learning a robust argumentation and deploying our attack connected a existent robot," Garg explained. "First, we created a simulation situation corresponding to the real-world script we were trying to solve."
The simulated situation was created utilizing NVIDIA's precocious released Isaac Gym Simulator. This simulator tin execute highly realistic simulations, leveraging the powerfulness of NVIDIA GPUs. By utilizing the Isaac Gym platform, Garg and his colleagues were capable to importantly trim the magnitude of computations indispensable to construe dexterous manipulation skills from simulations to real-world settings, decreasing their system's requirements from a clump with hundreds of CPUs and aggregate GPUs to a azygous GPU.
"Reinforcement learning requires america to usage representations of variables successful our occupation due to solving the task," Garg said. "The Real Robot situation required competitors to repose cubes successful some presumption and orientation. This made the task importantly much challenging than erstwhile efforts, arsenic the learned neural web controller needed to beryllium capable to commercialized disconnected these 2 objectives."
To lick the entity manipulation occupation posed by the Real Robot challenge, Garg and his colleagues decided to usage 'keypoint representation," a mode of representing objects by focusing connected the main 'interest points' successful an image. These are points that stay unchanged irrespective of an image's size, rotation, distortions oregon different variations.
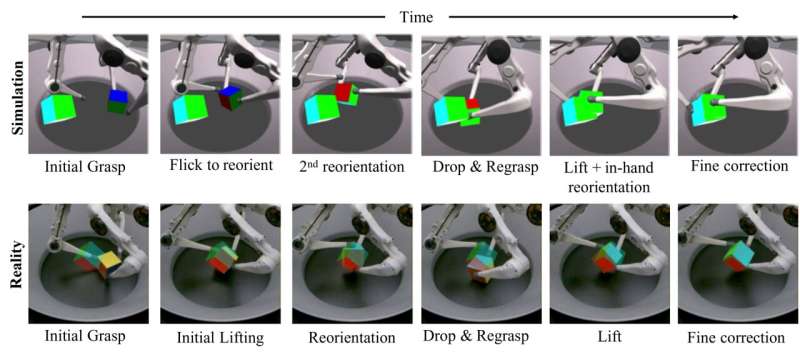
In their study, the researchers utilized keypoints to correspond the airs of a cube that the robot was expected to manipulate successful the representation information fed to their neural network. They besides utilized them to cipher the alleged reward function, which tin yet let reinforcement learning algorithms to amended their show implicit time.
"Finally, we added randomizations to the environment," Garg said. "These see randomizing the inputs to the network, the actions it takes, arsenic good arsenic assorted situation parameters specified arsenic the friction of the cube and adding random forces upon it. The effect of this is to unit the neural web controller to grounds behaviour which is robust to a scope of situation parameters."
The researchers trained their reinforcement learning exemplary successful the simulated situation they created utilizing Isaac Gym, implicit the people of 1 day. In simulation, the algorithm was presented with 16,000 simulated robots, producing ~50,000 steps / 2nd of information that was past utilized to bid the network.
"The argumentation was past uploaded to the robot farm, wherever it was deployed connected a random robot from a excavation of aggregate akin robots," Garg said. "Here, the argumentation does not get re-trained based connected each robot's unsocial parameters—it is already capable to accommodate to them. After the manipulation task is completed, the information is uploaded to beryllium accessed by the researchers."
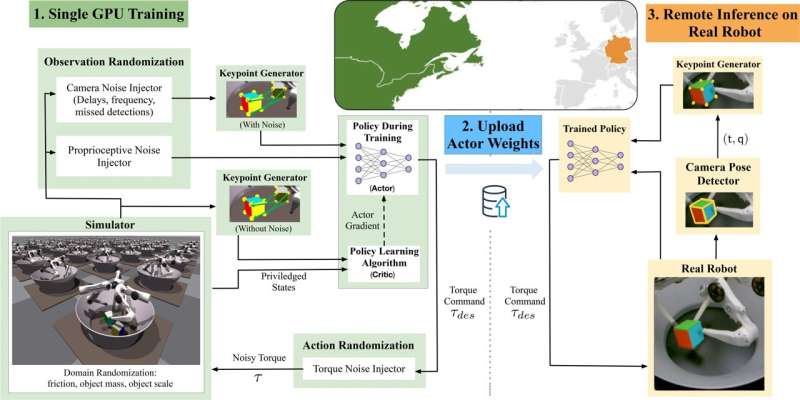
Garg and his colleagues were yet capable to efficaciously transportation the results achieved by their heavy reinforcement learning algorithm successful simulations to existent robots, with acold little computational powerfulness than different teams required successful the past. In addition, they demonstrated the effectual integration of highly parallel simulation tools with modern heavy reinforcement learning methods to efficaciously lick challenging dextrous manipulation tasks.
The researchers besides recovered that the usage of keypoint practice led to faster grooming and a higher occurrence complaint successful real-world tasks. In the future, the model they developed could assistance to accelerate probe astir dexterous manipulation and sim2real transfer, for lawsuit allowing researchers to make policies wholly successful simulation with mean computational resources and deploy them connected existent low-cost robots.
Credit: Allshire et al.
"We present anticipation to physique connected our model to proceed to beforehand the authorities of in-hand manipulation for much general-purpose manipulation beyond in-hand reposing," Garg said. "This enactment lays the instauration for america to survey the halfway concepts of the connection of manipulation, peculiarly tasks that impact nonstop grasping and entity reorientation ranging from opening h2o bottles to grasping java cups."
More information: Arthur Allshire et al, Transferring dexterous manipulation from GPU simulation to a distant real-world trifinger. arXiv:2108.09779v1 [cs.RO], arxiv.org/abs/2108.09779
© 2021 Science X Network
Citation: A strategy to transportation robotic dexterous manipulation skills from simulations to existent robots (2021, October 20) retrieved 20 October 2021 from https://techxplore.com/news/2021-10-robotic-dexterous-skills-simulations-real.html
This papers is taxable to copyright. Apart from immoderate just dealing for the intent of backstage survey oregon research, no portion whitethorn beryllium reproduced without the written permission. The contented is provided for accusation purposes only.