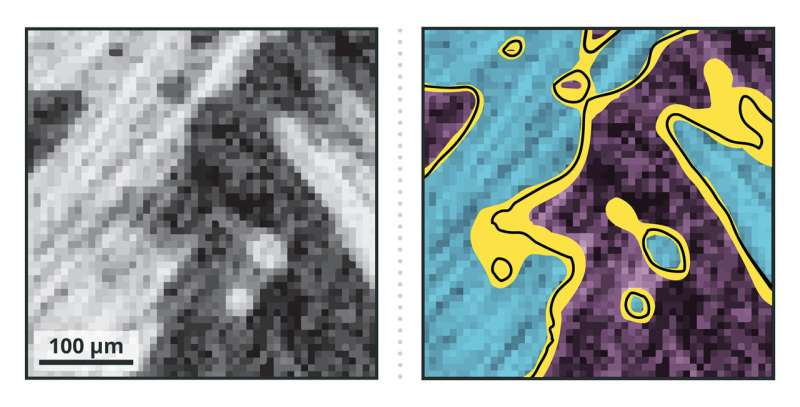
Sandia National Laboratories researchers person created a method of processing 3D images for machine simulations that could person beneficial implications for respective industries, including wellness care, manufacturing and electrical vehicles.
At Sandia, the method could beryllium captious successful certifying the credibility of high-performance computer simulations utilized successful determining the effectiveness of assorted materials for weapons programs and different efforts, said Scott A. Roberts, Sandia's main researcher connected the project. Sandia tin besides usage the caller 3D-imaging workflow to trial and optimize batteries utilized for large-scale vigor retention and successful vehicles.
"It's truly accordant with Sandia's ngo to bash credible, high-consequence machine simulation," helium said. "We don't privation to conscionable springiness you an reply and say, 'trust us.' We're going to say, 'here's our reply and here's however assured we are successful that answer,' truthful that you tin marque informed decisions."
The researchers shared the caller workflow, dubbed by the squad arsenic EQUIPS for Efficient Quantification of Uncertainty successful Image-based Physics Simulation, successful a insubstantial published contiguous successful the diary Nature Communications.
"This workflow leads to much reliable results by exploring the effect that ambiguous entity boundaries successful a scanned representation person successful simulations," said Michael Krygier, a Sandia postdoctoral appointee and pb writer connected the paper. "Instead of utilizing 1 mentation of that boundary, we're suggesting you request to execute simulations utilizing antithetic interpretations of the bound to scope a much informed decision."
EQUIPS tin usage instrumentality learning to quantify the uncertainty successful however an representation is drawn for 3D machine simulations. By giving a scope of uncertainty, the workflow allows decision-makers to see best- and worst-case outcomes, Roberts said.
Workflow EQUIPS decision-makers with amended information
Think of a doc examining a CT scan to make a crab attraction plan. That scan tin beryllium rendered into a 3D image, which tin past beryllium utilized successful a machine simulation to make a radiation dose that volition efficiently dainty a tumor without unnecessarily damaging surrounding tissue. Normally, the simulation would nutrient 1 effect due to the fact that the 3D representation was rendered once, said Carianne Martinez, a Sandia machine scientist.
But, drafting entity boundaries successful a scan tin beryllium hard and determination is much than 1 sensible mode to bash so, she said. "CT scans aren't cleanable images. It tin beryllium hard to spot boundaries successful immoderate of these images."
Humans and machines volition gully antithetic but tenable interpretations of the tumor's size and signifier from those blurry images, Krygier said.
Using the EQUIPS workflow, which tin usage instrumentality learning to automate the drafting process, the 3D representation is rendered into galore viable variations showing size and determination of a imaginable tumor. Those antithetic renderings volition nutrient a scope of antithetic simulation outcomes, Martinez said. Instead of 1 answer, the doc volition person a scope of prognoses to see that tin impact hazard assessments and attraction decisions, beryllium they chemotherapy oregon surgery.
"When you're moving with real-world information determination is not a single-point solution," Roberts said. "If I privation to beryllium truly assured successful an answer, I request to recognize that the worth tin beryllium anyplace betwixt 2 points, and I'm going to marque decisions based connected knowing it's determination successful this scope not conscionable reasoning it's astatine 1 point."
The EQUIPS squad has made the root codification and a moving illustration of the caller workflow disposable online for different researchers and programmers. Bayesian Convolutional Neural Network root codification is disposable here and the Monte Carlo Dropout Network root codification here. Both are connected GitHub. A python Jupyter notebook demonstrating the full EQUIPS workflow connected a elemental manufactured representation is disposable here.
It's a question of segmentation
The archetypal measurement of image-based simulation is the representation segmentation, oregon enactment simply, deciding which pixel (voxel successful a 3D image) to delegate to each entity and truthful drafting the bound betwixt 2 objects. From there, scientists tin statesman to physique models for computational simulation. But pixels and voxels volition blend with gradual gradient changes, truthful it is not ever wide wherever to gully the bound line—the grey areas successful a achromatic and achromatic CT scan oregon X-ray, Krygier said.
The inherent occupation with segmenting a scanned representation is that whether it's done by a idiosyncratic utilizing the champion bundle tools disposable oregon with the latest successful instrumentality learning capabilities, determination are galore plausible ways to delegate the pixels to the objects, helium said.
Two radical performing segmentation connected the aforesaid representation are apt to take a antithetic operation of filtering and techniques starring to antithetic but inactive valid segmentations. There is nary crushed to favour 1 representation segmentation implicit another. It's the aforesaid with precocious instrumentality learning techniques. While it tin beryllium quicker, much accordant and much close than manual segmentation, antithetic machine neural networks usage varying inputs and enactment connected antithetic parameters. Therefore, they tin nutrient antithetic but inactive valid segmentations, Martinez said.
Sandia's EQUIPS workflow does not destruct specified segmentation uncertainty, but it improves the credibility of the last simulations by making the antecedently unrecognized uncertainty disposable to the decision-maker, Krygier said.
EQUIPS tin employment 2 types of instrumentality learning techniques—Monte Carlo Dropout Networks and Bayesian Convolutional Neural Networks—to execute representation segmentation, with some approaches creating a acceptable of representation segmentation samples. These samples are combined to representation the probability that a definite pixel oregon voxel is successful the segmented material. To research the interaction of segmentation uncertainty, EQUIPS creates a probability representation to get segmentations, which are past utilized to execute aggregate simulations and cipher uncertainty distributions.
Funded by Sandia's Laboratory Directed Research and Development program, the probe was conducted with partners astatine Indiana-based Purdue University, a subordinate of the Sandia Academic Alliance Program. Researchers person made the source code and an EQUIPS workflow illustration disposable online.
To exemplify the divers applications that tin payment from the EQUIPS workflow, the researchers demonstrated successful the Nature Communications insubstantial respective uses for the caller method: CT scans of graphite electrodes successful lithium-ion batteries, astir commonly recovered successful electrical vehicles, computers, aesculapian instrumentality and aircraft; a scan of a woven composite being tested for thermal extortion connected atmospheric reentry vehicles, specified arsenic a rocket oregon a missile; and scans of some the quality aorta and spine.
"What we truly person done is accidental that you tin instrumentality instrumentality learning segmentation and not lone conscionable driblet that successful and get a azygous reply out, but you tin objectively probe that instrumentality learning segmentation to look astatine that ambiguity oregon uncertainty," Roberts said. "Coming up with the uncertainty makes it much credible and gives much accusation to those needing to marque decisions, whether successful engineering, wellness attraction oregon different fields wherever high-consequence machine simulations are needed."
More information: Michael C. Krygier et al, Quantifying the chartless interaction of segmentation uncertainty connected image-based simulations, Nature Communications (2021). DOI: 10.1038/s41467-021-25493-8
Citation: A 3D-imaging workflow has benefits for medicine, electrical cars and atomic deterrence (2021, September 14) retrieved 14 September 2021 from https://techxplore.com/news/2021-09-3d-imaging-workflow-benefits-medicine-electric.html
This papers is taxable to copyright. Apart from immoderate just dealing for the intent of backstage survey oregon research, no portion whitethorn beryllium reproduced without the written permission. The contented is provided for accusation purposes only.